Trainable piecewise linear activation function generation method
An activation function and linear function technology, applied in the computer field, can solve the problems of slow hardware implementation, lack of computing resources, and no solution to nonlinear mapping, and achieve the effect of speeding up the operation speed and simple linear operation conditions.
- Summary
- Abstract
- Description
- Claims
- Application Information
AI Technical Summary
Problems solved by technology
Method used
Image
Examples
Embodiment Construction
[0025] The present invention will be described in detail below in conjunction with the accompanying drawings.
[0026] This implementation case uses the approximation of the tanh function as an example, and discloses a method for generating a trainable piecewise linear activation function. The process is as follows figure 1 As shown, the steps are as follows:
[0027] Step 1) Determine that the non-linear function to be replaced is the tanh function.
[0028] Segment the selected nonlinear function. In this implementation case, the nonlinear activation function is divided into eight segments, and the negative semi-axis is divided into four segments, respectively (-∞,-6], (-6,-4], (-4 ,-2], (-2,0], four segments of the positive semi-axis, respectively (0,2], (2,4], (4,6], (6,+∞), positive and negative semi-axis with The y-axis is the center and is axisymmetric.
[0029] Step 2) Analyze the non-linear activation function divided into eight sections separately, and analyze the...
PUM
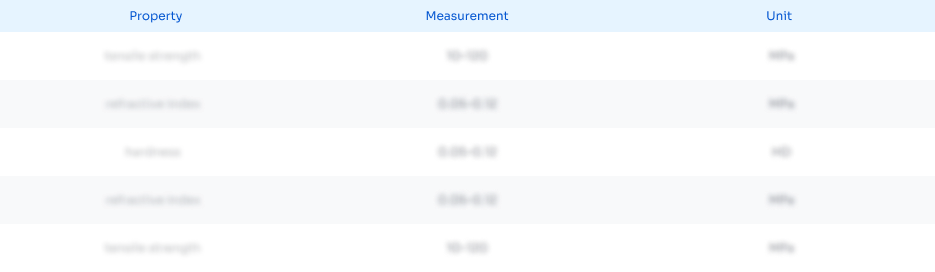
Abstract
Description
Claims
Application Information
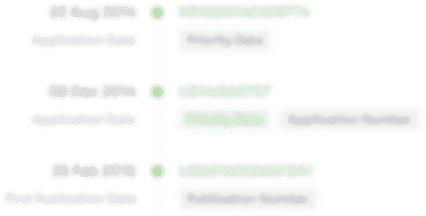
- R&D Engineer
- R&D Manager
- IP Professional
- Industry Leading Data Capabilities
- Powerful AI technology
- Patent DNA Extraction
Browse by: Latest US Patents, China's latest patents, Technical Efficacy Thesaurus, Application Domain, Technology Topic, Popular Technical Reports.
© 2024 PatSnap. All rights reserved.Legal|Privacy policy|Modern Slavery Act Transparency Statement|Sitemap|About US| Contact US: help@patsnap.com