Hyperspectral Image Classification Method Based on Multi-class Generative Adversarial Network
A hyperspectral image and classification method technology, applied in the field of image processing and image classification, can solve the problems of too few numbers, network overfitting, and low classification accuracy, so as to improve classification accuracy, improve accuracy, and enhance The effect of the ability to extract features
- Summary
- Abstract
- Description
- Claims
- Application Information
AI Technical Summary
Problems solved by technology
Method used
Image
Examples
Embodiment Construction
[0040] The present invention will be further described below in conjunction with the accompanying drawings.
[0041] combined with figure 1 Among them, the concrete steps that realize the present invention are as follows:
[0042] Step 1, input hyperspectral image.
[0043] Step 2, get the sample set.
[0044] Center each labeled pixel in the hyperspectral image to delineate a 27×27 pixel-sized spatial window.
[0045] All the pixels in each spatial window form a data cube.
[0046] Combine all data cubes into a sample set of hyperspectral images.
[0047] Step 3, generate training samples and test samples.
[0048] In the hyperspectral image sample set, 5% of the samples are randomly selected to form the hyperspectral image training samples; the remaining 95% of the samples are used to form the hyperspectral image test samples.
[0049] Step 4, build a multi-class generative confrontation network.
[0050] Build a generator consisting of a fully connected layer and 4 d...
PUM
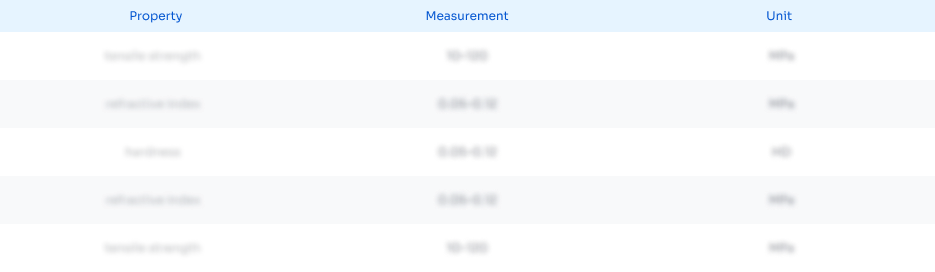
Abstract
Description
Claims
Application Information
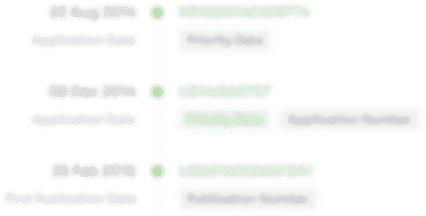
- R&D Engineer
- R&D Manager
- IP Professional
- Industry Leading Data Capabilities
- Powerful AI technology
- Patent DNA Extraction
Browse by: Latest US Patents, China's latest patents, Technical Efficacy Thesaurus, Application Domain, Technology Topic, Popular Technical Reports.
© 2024 PatSnap. All rights reserved.Legal|Privacy policy|Modern Slavery Act Transparency Statement|Sitemap|About US| Contact US: help@patsnap.com