Fault classification model and method based on stacked sparse Gaussian Bernoulli restricted Boltzmann machine and reinforcement learning
A technology limited to Boltzmann machines and fault classification, applied in neural learning methods, character and pattern recognition, biological neural network models, etc., can solve the problems of few label samples and strong correlation, and achieve the effect of improving accuracy
- Summary
- Abstract
- Description
- Claims
- Application Information
AI Technical Summary
Problems solved by technology
Method used
Image
Examples
Embodiment Construction
[0030] The fault classification method based on the stacked sparse Gaussian Bernoulli Restricted Boltzmann Machine and reinforcement learning of the present invention will be further described in detail below in combination with specific embodiments.
[0031] A fault classification method based on stacked sparse Gaussian Bernoulli Restricted Boltzmann Machines and reinforcement learning, where,
[0032] The stacked sparse Gaussian Bernoulli restricted Boltzmann machine model is divided into four layers, the first layer is an input layer, the second and third layers are hidden layers, and the fourth layer is a category layer, wherein the first layer and The second layer constitutes a sparse Gauss-Bernoulli restricted Boltzmann machine, that is, SGRBM, and the second layer and the third and fourth layers constitute a sparse category Gauss-Bernoulli-restricted Boltzmann machine, namely SCGRBM, After stacking, the sparse deep belief network is formed; the parameters related to the...
PUM
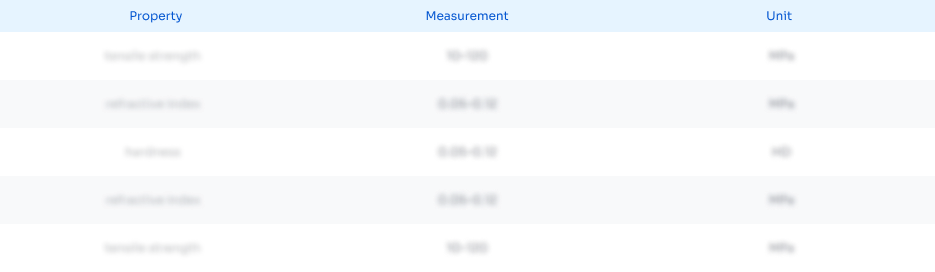
Abstract
Description
Claims
Application Information
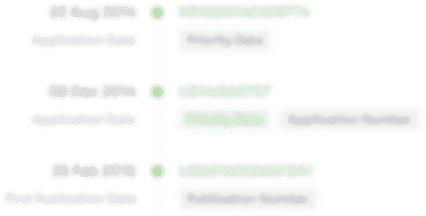
- R&D Engineer
- R&D Manager
- IP Professional
- Industry Leading Data Capabilities
- Powerful AI technology
- Patent DNA Extraction
Browse by: Latest US Patents, China's latest patents, Technical Efficacy Thesaurus, Application Domain, Technology Topic, Popular Technical Reports.
© 2024 PatSnap. All rights reserved.Legal|Privacy policy|Modern Slavery Act Transparency Statement|Sitemap|About US| Contact US: help@patsnap.com