Method, system and related equipment for scheduling deep learning jobs
A deep learning and job scheduling technology, applied in the field of artificial intelligence, which can solve the problems of different scheduling requirements of typical system services, lack of batch abstraction and mechanism, and reduced user experience.
- Summary
- Abstract
- Description
- Claims
- Application Information
AI Technical Summary
Problems solved by technology
Method used
Image
Examples
Embodiment approach
[0090] In the first way, the job request also includes at least one of the following information: job name, deep learning program storage location, application startup file, data set storage location, the type of the at least one task, the at least one The quantity of each task in the tasks, the command line parameters of the job, and the resource requirements of each task in the at least one task.
[0091] In the second way, the job request also includes at least one of the following information: job name, deep learning program, application startup file, data set storage location, type of the at least one task, and The quantity of each type of task, job command line parameters, and resource requirements of each task in the at least one task.
[0092] Among them, the job name is the identifier of the deep learning job. The storage location of the deep learning program is used for the computing node to read the deep learning program according to the storage location of the app...
PUM
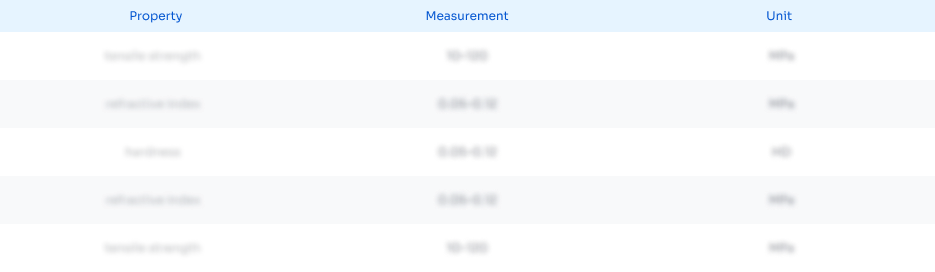
Abstract
Description
Claims
Application Information
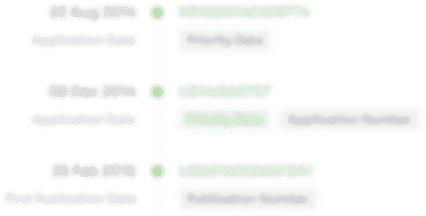
- R&D
- Intellectual Property
- Life Sciences
- Materials
- Tech Scout
- Unparalleled Data Quality
- Higher Quality Content
- 60% Fewer Hallucinations
Browse by: Latest US Patents, China's latest patents, Technical Efficacy Thesaurus, Application Domain, Technology Topic, Popular Technical Reports.
© 2025 PatSnap. All rights reserved.Legal|Privacy policy|Modern Slavery Act Transparency Statement|Sitemap|About US| Contact US: help@patsnap.com