Residual SSD model-based traffic sign detection and recognition method
A recognition method and technology for traffic signs, applied in character and pattern recognition, biological neural network models, instruments, etc., can solve the problems of large proportion of traffic signs, complex traffic, and inability to meet the needs of traffic scene detection, and improve the possibility of and speed effects
- Summary
- Abstract
- Description
- Claims
- Application Information
AI Technical Summary
Problems solved by technology
Method used
Image
Examples
Embodiment approach
[0088] Embodiments of the present invention are described as follows:
[0089] 1. Data preprocessing
[0090] The ratio of the number of training and test pictures in the Tsinghua-Tencent 100K dataset is roughly 2:1. Select 45 signs with more than 100 occurrences as targets for detection and recognition, and use the bounding box coordinates and categories as labels for detection and recognition, respectively.
[0091] 2. Experimental environment
[0092] The training and testing of the model are carried out on the Linux PC side, including an Intel i7-7700K CPU with 32GB memory and two NVIDIA GeForce GTX 1080Ti GPUs with 11G memory.
[0093] 3. Learning parameter configuration
[0094] The initial learning rate of the training is set to 0.001, which drops to 0.0001 after 40,000 iterations, and then continues to iterate for 40,000 iterations with a learning rate of 0.0001 to stop. Use a momentum of 0.9 and a decay rate of 0.0005. Experiments are performed on the CAFFE archi...
PUM
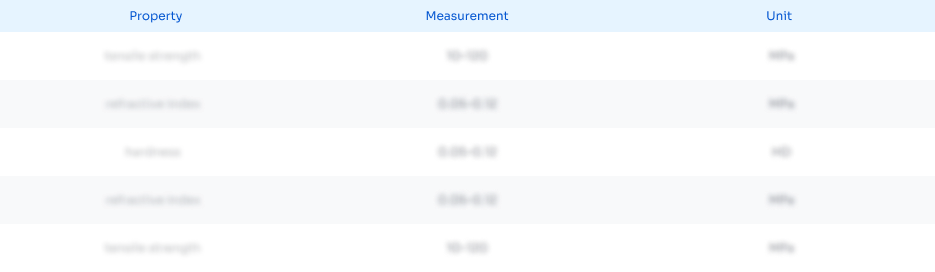
Abstract
Description
Claims
Application Information
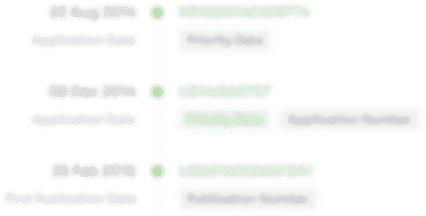
- R&D
- Intellectual Property
- Life Sciences
- Materials
- Tech Scout
- Unparalleled Data Quality
- Higher Quality Content
- 60% Fewer Hallucinations
Browse by: Latest US Patents, China's latest patents, Technical Efficacy Thesaurus, Application Domain, Technology Topic, Popular Technical Reports.
© 2025 PatSnap. All rights reserved.Legal|Privacy policy|Modern Slavery Act Transparency Statement|Sitemap|About US| Contact US: help@patsnap.com