Single channel-based non-supervision target speaker speech extraction method
A speech extraction and unsupervised technology, which is applied in the directions of speech analysis, speech recognition, character and pattern recognition, etc., can solve problems such as the inability to guarantee the accuracy of clustering, the difficulty in selecting the characteristics of the human ear model, and the large amount of computation. , to achieve the effect of improving adaptability and intelligence
- Summary
- Abstract
- Description
- Claims
- Application Information
AI Technical Summary
Problems solved by technology
Method used
Image
Examples
Embodiment Construction
[0072] In order to make the object, technical solution and advantages of the present invention clearer, the present invention will be further described in detail below in conjunction with the accompanying drawings.
[0073] refer to figure 1 As shown, a single-channel, unsupervised target speaker voice extraction method of the present invention includes a teacher's language detection step and a teacher's language GGMM model training step.
[0074] Such as figure 2 As shown, teacher language detection should include the following steps:
[0075] S110, recording;
[0076] S120, voice signal preprocessing;
[0077] S130. Speech segmentation and modeling;
[0078] S140. Teacher voice detection.
[0079] Such as image 3 As shown, the teacher's voice GGMM model training functional unit should include the following steps:
[0080] S110, recording;
[0081] S120, voice signal preprocessing;
[0082] S130. Speech segmentation and modeling;
[0083] S240, clustering.
[008...
PUM
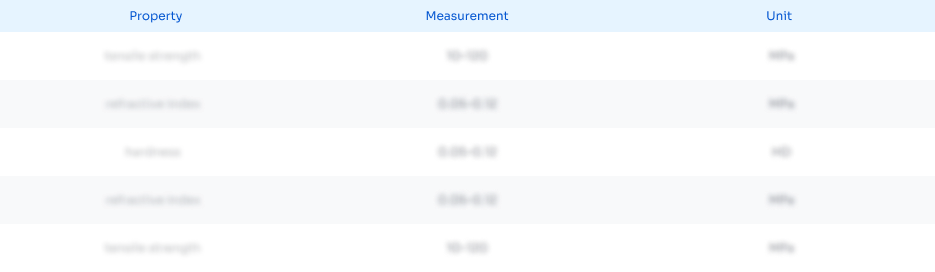
Abstract
Description
Claims
Application Information
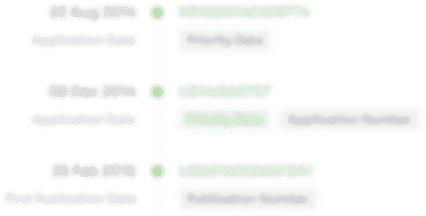
- R&D
- Intellectual Property
- Life Sciences
- Materials
- Tech Scout
- Unparalleled Data Quality
- Higher Quality Content
- 60% Fewer Hallucinations
Browse by: Latest US Patents, China's latest patents, Technical Efficacy Thesaurus, Application Domain, Technology Topic, Popular Technical Reports.
© 2025 PatSnap. All rights reserved.Legal|Privacy policy|Modern Slavery Act Transparency Statement|Sitemap|About US| Contact US: help@patsnap.com