An Image Denoising Method Based on Adaptive Weighted Total Variational Model
A technology of full variational model and adaptive weight, applied in the field of image processing, which can solve the problems of noise sensitivity, lack of anti-diffusion ability, and affecting image denoising effect, so as to avoid the effect of ladder effect
- Summary
- Abstract
- Description
- Claims
- Application Information
AI Technical Summary
Problems solved by technology
Method used
Image
Examples
Embodiment 1
[0094] This embodiment chooses as Figure 4 As shown in the Lena (256×256) grayscale image, after adding Gaussian noise (σ=15) to the Lena (256×256) grayscale image, the following Figure 5 The noise image shown, for Figure 5 The noise image is denoised using the denoising method of the present invention, specifically according to the following steps:
[0095] Step 1, in order to reduce the sensitivity of the adaptive paradigm parameter g to noise, use guided filtering to process the noisy image, and get as follows Image 6 As shown in the smooth image, after the smooth image is processed by shock filter to enhance the edge information of the image, the following is obtained: Figure 7 The edge information enhanced image shown;
[0096] In order to reduce the sensitivity of the adaptive parameters to noise, the noisy image is processed with a non-local mean filter, and the following is obtained: Figure 8 The estimated denoised image u is shown as NL :
[0097]
[00...
PUM
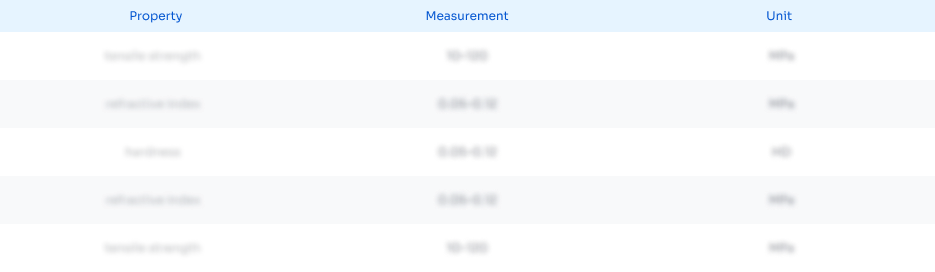
Abstract
Description
Claims
Application Information
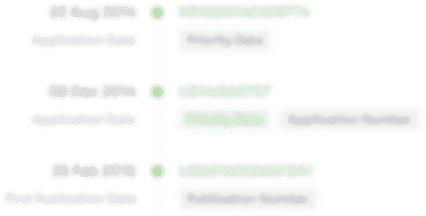
- R&D Engineer
- R&D Manager
- IP Professional
- Industry Leading Data Capabilities
- Powerful AI technology
- Patent DNA Extraction
Browse by: Latest US Patents, China's latest patents, Technical Efficacy Thesaurus, Application Domain, Technology Topic, Popular Technical Reports.
© 2024 PatSnap. All rights reserved.Legal|Privacy policy|Modern Slavery Act Transparency Statement|Sitemap|About US| Contact US: help@patsnap.com