A malicious code classification method based on multiple features and feature selection
A malicious code and feature selection technology, which is applied in the field of malicious code classification, can solve the problems of reduced training speed, achieve good classification performance, improve classification accuracy, and speed up training
- Summary
- Abstract
- Description
- Claims
- Application Information
AI Technical Summary
Problems solved by technology
Method used
Image
Examples
example 1
[0061] The specific implementation steps of this embodiment 1 are as follows: figure 1 As shown, the method includes the following steps:
[0062] Step A: Malicious code file preprocessing;
[0063] In this embodiment, the malicious code samples used are the datasets provided by Microsoft that include '.byte' files and '.asm' files, and the PE file headers of each malicious code sample have been removed;
[0064] Specifically in this embodiment, check the files in the sample set, and delete malicious code samples that only contain '.bytes' files or only '.asm' files;
[0065] Step B: Generate malicious code images and extract pixel features;
[0066] Malicious code pixel feature extraction process is as follows: figure 2 ;
[0067] Specific to this embodiment, use '.asm' file to generate malicious code image, use python to carry out feature extraction;
[0068] First read the '.asm' file and convert it to a hexadecimal file, and then split the hexadecimal string by byte....
PUM
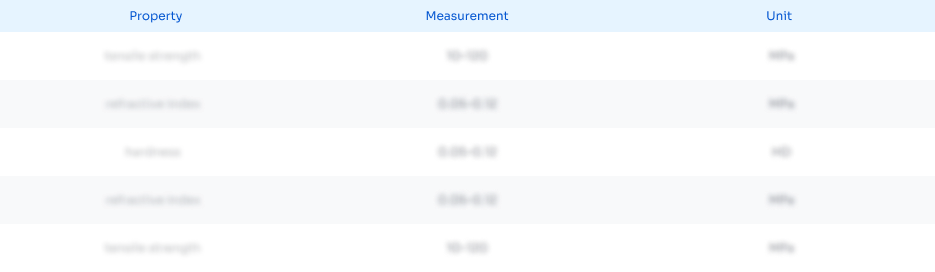
Abstract
Description
Claims
Application Information
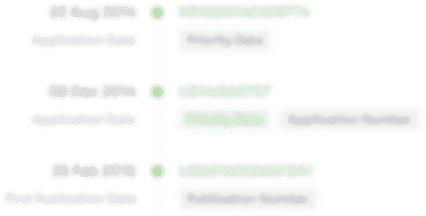
- R&D Engineer
- R&D Manager
- IP Professional
- Industry Leading Data Capabilities
- Powerful AI technology
- Patent DNA Extraction
Browse by: Latest US Patents, China's latest patents, Technical Efficacy Thesaurus, Application Domain, Technology Topic.
© 2024 PatSnap. All rights reserved.Legal|Privacy policy|Modern Slavery Act Transparency Statement|Sitemap