A Weld Defect Recognition Method Based on Improved Convolutional Neural Network
A technology of convolutional neural network and defect identification, applied in the field of automatic identification of weld defects, to achieve a high rate of correct defect recognition
- Summary
- Abstract
- Description
- Claims
- Application Information
AI Technical Summary
Problems solved by technology
Method used
Image
Examples
Embodiment Construction
[0037] The invention provides a weld defect recognition method based on an improved convolutional neural network, and constructs a convolutional neural network with a specific architecture. The specific architecture is to propose a comprehensive consideration of the pooling domain and its feature map features in the pooling layer Distributed pooling model, which is based on the classic maximum pooling model, according to the pooling domain and its feature map feature distribution, introduces a correction factor μ to correct the maximum pooling feature; proposes a traditional feature evaluation Method A feature selection method combining Relief algorithm and neural network; minimize the cost function and iterate with the goal of training to form the weld defect recognition neural network, and send the image of the weld to be recognized into the trained deep neural network to realize Identification of weld defect types. The invention avoids the process of manually extracting fea...
PUM
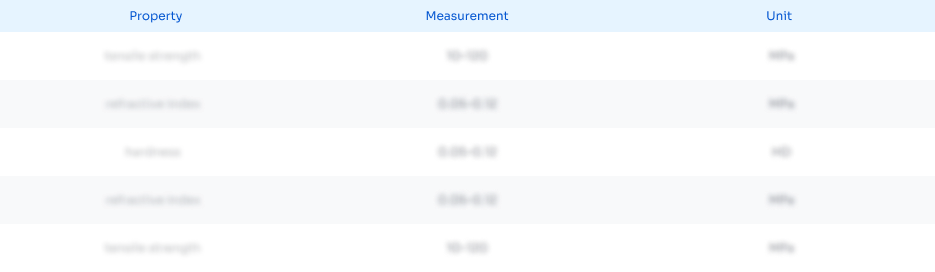
Abstract
Description
Claims
Application Information
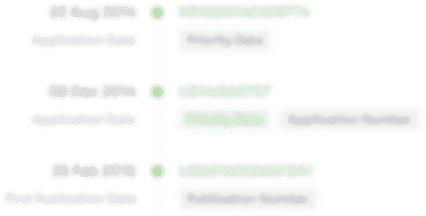
- R&D Engineer
- R&D Manager
- IP Professional
- Industry Leading Data Capabilities
- Powerful AI technology
- Patent DNA Extraction
Browse by: Latest US Patents, China's latest patents, Technical Efficacy Thesaurus, Application Domain, Technology Topic, Popular Technical Reports.
© 2024 PatSnap. All rights reserved.Legal|Privacy policy|Modern Slavery Act Transparency Statement|Sitemap|About US| Contact US: help@patsnap.com