A sparse code division multiple access signal detection method based on depth neural network
A deep neural network and sparse code division multiple access technology, applied in the field of multi-user detection, can solve problems such as high computational complexity and FPGA resource consumption, and achieve the effect of reducing computational pressure, principles and implementation methods.
- Summary
- Abstract
- Description
- Claims
- Application Information
AI Technical Summary
Benefits of technology
Problems solved by technology
Method used
Image
Examples
Embodiment Construction
[0047] The present invention will be further described below in conjunction with the accompanying drawings.
[0048]The complex signals of multiple users are superimposed on multiple resource blocks, and the number of users is greater than the number of resources. At this time, information of multiple users is carried on one resource block. The traditional solution is to use the belief propagation algorithm to iterate the information on the edges of the factor graph, and the algorithm converges after several iterations. However, the MPA algorithm based on this principle has high computational complexity and consumes a lot of FPGA resources. To this end, this method provides a SCMA detection algorithm based on a deep neural network, which transfers the consumption of computing power to the currently idle AI chip, and through parameter training, this method also obtains a small amount of performance improvement. This method specifically comprises the following steps:
[0049] (...
PUM
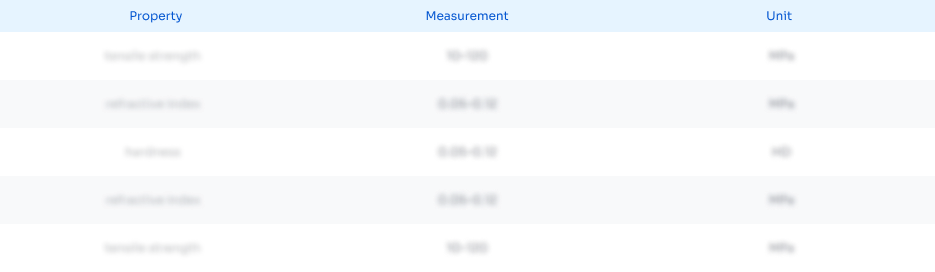
Abstract
Description
Claims
Application Information
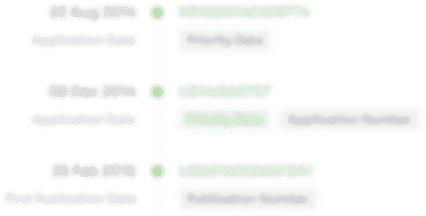
- R&D Engineer
- R&D Manager
- IP Professional
- Industry Leading Data Capabilities
- Powerful AI technology
- Patent DNA Extraction
Browse by: Latest US Patents, China's latest patents, Technical Efficacy Thesaurus, Application Domain, Technology Topic.
© 2024 PatSnap. All rights reserved.Legal|Privacy policy|Modern Slavery Act Transparency Statement|Sitemap