Aircraft system fault diagnosis method based on MSCNN deep learning
A deep learning and aircraft system technology, applied in the testing of mechanical components, testing of machine/structural components, measuring devices, etc., can solve problems such as incomplete expression of the diagnosed system, and achieve accurate results
- Summary
- Abstract
- Description
- Claims
- Application Information
AI Technical Summary
Problems solved by technology
Method used
Image
Examples
Embodiment Construction
[0069] In order to make the technical means, creative features, goals and effects achieved by the present invention easy to understand, the present invention will be further described below in conjunction with specific embodiments.
[0070] see figure 1 , figure 2 with image 3 , a kind of aircraft system fault diagnosis method based on MSCNN deep learning of the present invention, comprises the steps:
[0071] S1, collecting decoded aircraft QAR data;
[0072] The typical variables that can best reflect the working state of the aircraft system are selected as the input characteristic data of the model, and the threshold ranges of different working conditions are analyzed.
[0073] S2, transforming the aircraft state parameters in the aircraft QAR data into fixed-size two-dimensional data;
[0074] S3, establish the deep learning model MSCNN of the whole task profile;
[0075] S4, automatically identify the working conditions according to the samples and adaptively gener...
PUM
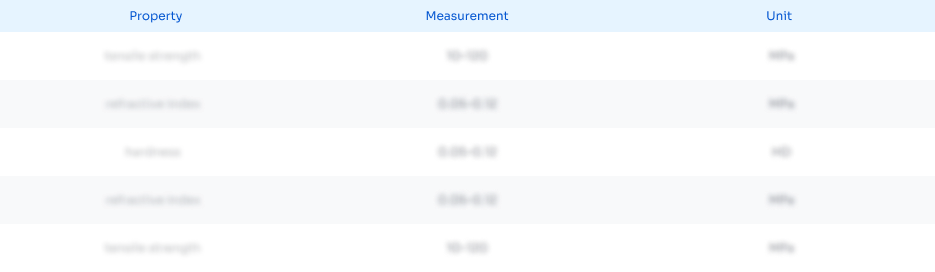
Abstract
Description
Claims
Application Information
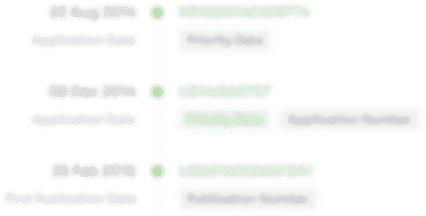
- R&D
- Intellectual Property
- Life Sciences
- Materials
- Tech Scout
- Unparalleled Data Quality
- Higher Quality Content
- 60% Fewer Hallucinations
Browse by: Latest US Patents, China's latest patents, Technical Efficacy Thesaurus, Application Domain, Technology Topic, Popular Technical Reports.
© 2025 PatSnap. All rights reserved.Legal|Privacy policy|Modern Slavery Act Transparency Statement|Sitemap|About US| Contact US: help@patsnap.com