Prediction method of urban PM10 concentration based on characteristic expansion fusion neural network
A neural network and concentration prediction technology, which is applied in the direction of measuring devices, suspension and porous material analysis, particle suspension analysis, etc., can solve the problems of missing features, insufficient feature dimensions, and inability to predict the concentration of pollutants, so as to achieve accurate prediction and solution. incomplete effect
- Summary
- Abstract
- Description
- Claims
- Application Information
AI Technical Summary
Problems solved by technology
Method used
Image
Examples
Embodiment Construction
[0060] The present invention will be described in detail below in conjunction with the accompanying drawings and specific embodiments. This embodiment is carried out on the premise of the technical solution of the present invention, and detailed implementation and specific operation process are given, but the protection scope of the present invention is not limited to the following embodiments.
[0061] First define the air pollutant concentration prediction:
[0062] Definition 1 Prediction of air pollutant concentration: mainly through historical pollutants and meteorological information, to predict the concentration of a series of air pollution such as PM2.5 and PM10 in a certain period of time in the future. It is one of the key research topics, so it has certain interdisciplinary nature.
[0063] Definition 2 Traditional forecasting methods: non-deep learning air pollutant concentration forecasting methods are collectively referred to as traditional forecasting methods, ...
PUM
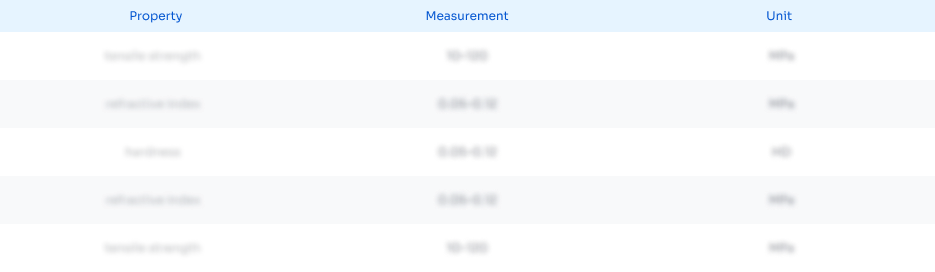
Abstract
Description
Claims
Application Information
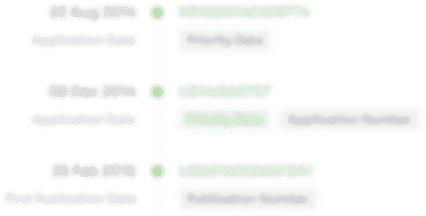
- Generate Ideas
- Intellectual Property
- Life Sciences
- Materials
- Tech Scout
- Unparalleled Data Quality
- Higher Quality Content
- 60% Fewer Hallucinations
Browse by: Latest US Patents, China's latest patents, Technical Efficacy Thesaurus, Application Domain, Technology Topic, Popular Technical Reports.
© 2025 PatSnap. All rights reserved.Legal|Privacy policy|Modern Slavery Act Transparency Statement|Sitemap|About US| Contact US: help@patsnap.com