Nonlinear dynamic process monitoring method based on canonical variable nonlinear principal component analysis
A nonlinear principal component and nonlinear dynamic technology, which is applied in the field of nonlinear dynamic process monitoring based on explicit polynomial mapping, can solve problems such as redundancy and low efficiency of infinite-dimensional nonlinear mapping, and achieve high fault detection rate and low False alarm rate and impact reduction effect
- Summary
- Abstract
- Description
- Claims
- Application Information
AI Technical Summary
Problems solved by technology
Method used
Image
Examples
Embodiment Construction
[0043] The technical solutions of the present invention will be further described in detail below in conjunction with specific embodiments.
[0044] figure 1 It is a schematic diagram of the principle of the monitoring method of the present invention. The proposed nonlinear dynamic process monitoring method mainly includes three stages. Stage 1, use CVA to reduce the influence of data dynamic characteristics; stage 2, map the state vector to high-dimensional feature space by displaying polynomial mapping; stage 3, use PCA to determine the first k pivots and remaining residuals, and calculate T 2 and Q c Statistics.
[0045] 1) Dynamic data preprocessing
[0046] Canonical variable analysis is a linear dimensionality reduction method based on multivariate statistical analysis, where the past observation vector y p,r and the future observation vector y f,r It is composed of the measured values of past and future p sampling moments in the data matrix Y respectively:
[0...
PUM
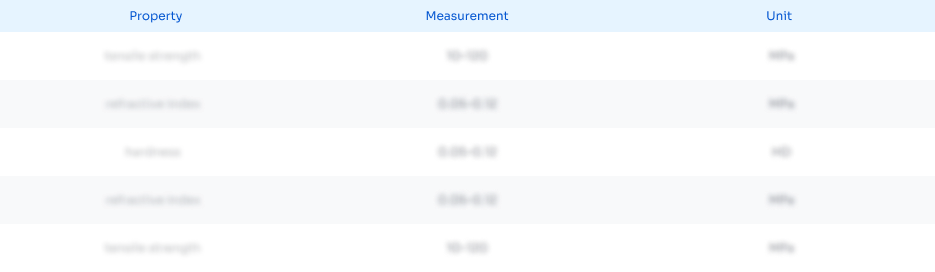
Abstract
Description
Claims
Application Information
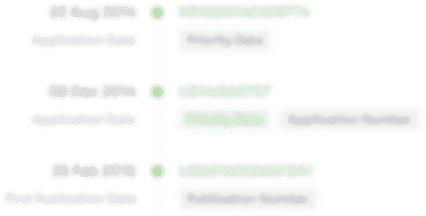
- R&D Engineer
- R&D Manager
- IP Professional
- Industry Leading Data Capabilities
- Powerful AI technology
- Patent DNA Extraction
Browse by: Latest US Patents, China's latest patents, Technical Efficacy Thesaurus, Application Domain, Technology Topic, Popular Technical Reports.
© 2024 PatSnap. All rights reserved.Legal|Privacy policy|Modern Slavery Act Transparency Statement|Sitemap|About US| Contact US: help@patsnap.com