An adversarial sample detection method based on the distance from a sample to a decision boundary
A technology against samples and detection methods, applied to instruments, character and pattern recognition, computer components, etc., can solve the problems of artificial intelligence classifier security vulnerabilities and unsatisfactory results, and achieve improved security and good detection results , the effect is obvious
- Summary
- Abstract
- Description
- Claims
- Application Information
AI Technical Summary
Problems solved by technology
Method used
Image
Examples
Embodiment Construction
[0021] Such as figure 1 As shown, this embodiment adopts the BelgiumTS data set, and protects the road sign recognition API from adversarial sample attacks through the method of this embodiment.
[0022] This embodiment specifically includes:
[0023] Step 1. Generation of adversarial samples: Use the API training sample set as normal samples, and use some of the normal samples to generate adversarial samples (mixed in equal proportions) through four attack methods: iter-FGSM, C&W, DeepFool, and JSMA.
[0024] Step 2. Elimination of invalid samples: Eliminate invalid samples for normal samples and adversarial samples: invalid samples include: ① they are normal samples, but are misidentified by the API, and these samples are close to the decision boundary, so they are eliminated; ② they are adversarial samples , but is correctly identified by the API, this type of adversarial sample attack fails and cannot threaten the API.
[0025] Step 3. Calculate the upper and lower bound...
PUM
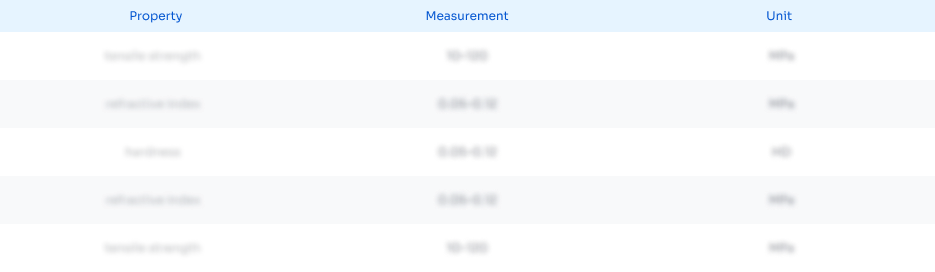
Abstract
Description
Claims
Application Information
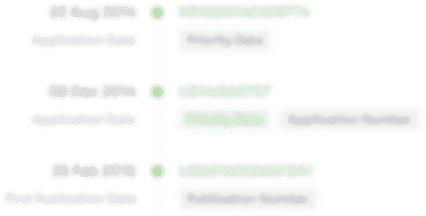
- R&D Engineer
- R&D Manager
- IP Professional
- Industry Leading Data Capabilities
- Powerful AI technology
- Patent DNA Extraction
Browse by: Latest US Patents, China's latest patents, Technical Efficacy Thesaurus, Application Domain, Technology Topic, Popular Technical Reports.
© 2024 PatSnap. All rights reserved.Legal|Privacy policy|Modern Slavery Act Transparency Statement|Sitemap|About US| Contact US: help@patsnap.com