Method for predicting remaining service life of rolling bearing integrated with KELM
A rolling bearing and life prediction technology, applied in the testing of mechanical components, testing of machine/structural components, measuring devices, etc., can solve problems such as low prediction accuracy, low prediction accuracy, and weak similarity
- Summary
- Abstract
- Description
- Claims
- Application Information
AI Technical Summary
Problems solved by technology
Method used
Image
Examples
Embodiment Construction
[0034] Such as figure 1 and figure 2 As shown, the specific implementation process of a KELM-integrated rolling bearing remaining service life prediction method described in this embodiment is as follows:
[0035] 1 Feature extraction and dimensionality reduction
[0036] 1.1 Raw vibration signal preprocessing
[0037] The collected original vibration signal is averaged to offset the DC component, and the least square method is used to eliminate the polynomial trend item of the vibration data. Then use the five-point cubic smoothing method to smooth the signal, as shown in formula (1), this method can reduce the high-frequency interference noise of the vibration signal and play a filtering role.
[0038]
[0039] In the formula, y i is the size of sequential sampling values (i=3,4,...,n-2), y i ’ is the size of the data value after smoothing, and n is the total number of data points.
[0040] The five-point cubic smoothing method uses the least square method to fit...
PUM
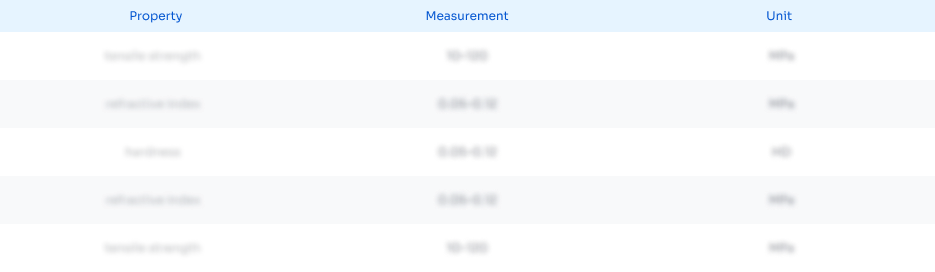
Abstract
Description
Claims
Application Information
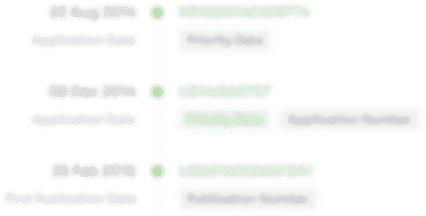
- R&D Engineer
- R&D Manager
- IP Professional
- Industry Leading Data Capabilities
- Powerful AI technology
- Patent DNA Extraction
Browse by: Latest US Patents, China's latest patents, Technical Efficacy Thesaurus, Application Domain, Technology Topic, Popular Technical Reports.
© 2024 PatSnap. All rights reserved.Legal|Privacy policy|Modern Slavery Act Transparency Statement|Sitemap|About US| Contact US: help@patsnap.com