A method and an apparatus for general image classification base on a semi-supervised generative adversarial network
A semi-supervised, network technology, applied in the field of deep learning, can solve problems such as large deformation, and achieve the effect of improving accuracy, generalization performance and classification accuracy
- Summary
- Abstract
- Description
- Claims
- Application Information
AI Technical Summary
Problems solved by technology
Method used
Image
Examples
example 1
[0079] Example 1 General image classification method based on semi-supervised generative confrontation network
[0080] Taking the MNIST handwritten digit data set as an example, according to the method of the present invention, compare the probability under the semi-supervised method and the supervised method, and the classification accuracy obtained is as shown in Table 1:
[0081]Table 1. Classification accuracy using semi-supervised learning and supervised learning on the MNIST dataset
[0082] Number of labeled images
[0083] In Table 1, the last row represents the classification accuracy obtained by the supervised learning method, and the remaining rows represent the classification accuracy obtained by the semi-supervised learning method of the present invention, and "the number of labeled images" refers to the original training data. 50,000 is the size of the MNIST training data set. In the experiment, keep the input size of supervised learning and semi-super...
example 2
[0084] Example 2 General image classification method based on semi-supervised generative confrontation network
[0085] Taking the CIFAR-10 data set as an example, according to the method of the present invention, comparing the probability under the semi-supervised method and the supervised method, the obtained classification accuracy is shown in Table 2:
[0086] Table 2. Classification accuracy using semi-supervised learning and supervised learning on the MNIST dataset
[0087]
[0088]
[0089] In Table 2, the last row represents the classification accuracy rate obtained by the supervised learning method, and the remaining rows represent the classification accuracy rate obtained by the semi-supervised learning method of the present invention. "The number of labeled images" refers to the original training data. 50,000 is the size of the CIFAR-10 training data set. In the experiment, keep the input size of supervised learning and semi-supervised learning equal to get th...
PUM
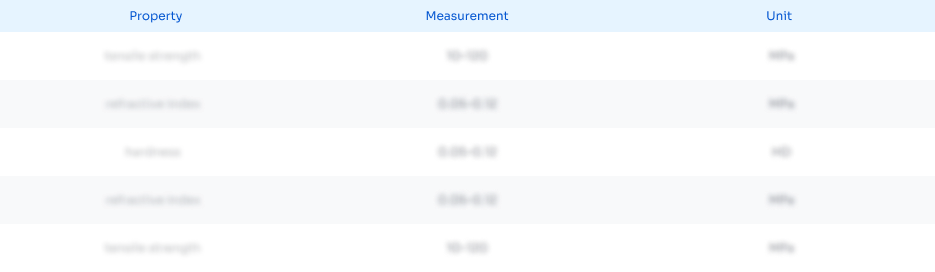
Abstract
Description
Claims
Application Information
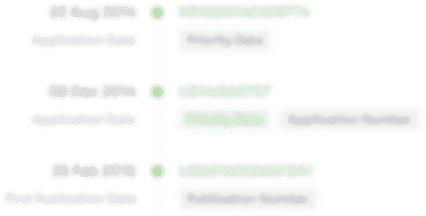
- Generate Ideas
- Intellectual Property
- Life Sciences
- Materials
- Tech Scout
- Unparalleled Data Quality
- Higher Quality Content
- 60% Fewer Hallucinations
Browse by: Latest US Patents, China's latest patents, Technical Efficacy Thesaurus, Application Domain, Technology Topic, Popular Technical Reports.
© 2025 PatSnap. All rights reserved.Legal|Privacy policy|Modern Slavery Act Transparency Statement|Sitemap|About US| Contact US: help@patsnap.com