Multi-Channel Network Predictive Optimization Method for Coronary Segmentation
A technology of network prediction and optimization methods, which is applied in image analysis, image enhancement, instruments, etc., can solve problems such as not being robust, and achieve the effect of increasing learning ability
- Summary
- Abstract
- Description
- Claims
- Application Information
AI Technical Summary
Problems solved by technology
Method used
Examples
Embodiment 1
[0018] This embodiment discloses a multi-channel network prediction optimization method for coronary artery segmentation, which includes the following steps:
[0019] S1. Using the CT image + ignoring the edge features in the Z direction as a sample, train the model Z;
[0020] S2. Input the CT image to be segmented and its edge features ignoring the Z direction into the model Z to obtain a first segmentation result.
Embodiment 2
[0022] S1. Using CT images and edge features in X, Y, and Z directions as samples, train model A.
[0023] S2. Input the CT image to be segmented and its edge features in the X, Y, and Z directions into the model A to obtain a first segmentation result.
[0024] Comparing the segmentation result of this embodiment with the segmentation result of Example 1, both models use CELoss as the loss function, and the final loss result: Example 1 is 0.018, and Example 2 is 0.03. It can be seen that the implementation The result precision obtained by the method adopted in Example 1 is obviously better than that in Example 2.
[0025] At the same time, the centerlines were extracted from the segmentation results of Example 1 and the segmentation results of Example 2, respectively, and the breakpoint detection was performed on the centerlines of the two respectively. In the final result, the number of breakpoints in Example 2 was significantly more than that in Example 1. of breaking poin...
Embodiment 3
[0027] Although the method of Embodiment 1 is used for segmentation, the accuracy is greatly improved, but there are still breakpoints unavoidably. Therefore, the present invention also repairs subsequent breakpoints.
[0028] S3. Using CT images + ignoring the edge features in the X direction and CT images + ignoring the edge features in the Y direction as samples, train the X model and the Y model respectively
[0029] S4. Perform centerline extraction on the first segmentation result;
[0030] S5. Performing break point detection on the extracted center line;
[0031] S6. Give an estimated direction to the breaking point according to the center point of the preceding sequence, and calculate the normal direction of the estimated direction;
[0032] S7. Calculate the components of the X direction and the Y direction in the normal direction of the estimated direction, take the direction with a large component as the main direction, and use the main direction as the neglected ...
PUM
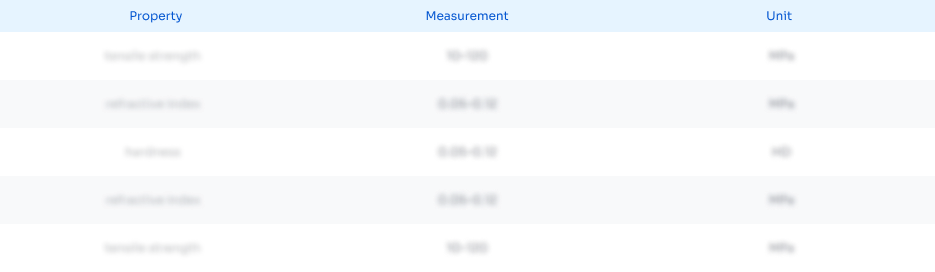
Abstract
Description
Claims
Application Information
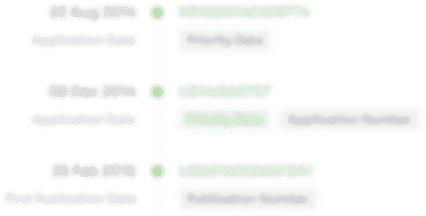
- R&D Engineer
- R&D Manager
- IP Professional
- Industry Leading Data Capabilities
- Powerful AI technology
- Patent DNA Extraction
Browse by: Latest US Patents, China's latest patents, Technical Efficacy Thesaurus, Application Domain, Technology Topic, Popular Technical Reports.
© 2024 PatSnap. All rights reserved.Legal|Privacy policy|Modern Slavery Act Transparency Statement|Sitemap|About US| Contact US: help@patsnap.com