A P300 detection method based on CNN-LSTM network
A detection method and network technology, applied in the field of EEG signal detection, can solve problems such as time-consuming, character recognition accuracy needs to be further improved, generalization ability and accuracy improvement, etc.
- Summary
- Abstract
- Description
- Claims
- Application Information
AI Technical Summary
Problems solved by technology
Method used
Image
Examples
Embodiment Construction
[0069] The present invention will be further described below in conjunction with specific examples.
[0070] Such as figure 1 As shown, the P300 detection method based on the CNN-LSTM network provided by the present embodiment collects EEG data through the P300 speller and performs preprocessing; then establishes an algorithm model and trains model parameters; finally tests the character recognition of the model Accuracy and other performance indicators, which include the following steps:
[0071] 1) Design the P300 character speller, collect EEG data and determine the training set and test set, including the following steps:
[0072] 1.1) Make sure to use figure 2 P3 speller for the BCI2000 platform shown, and determines how the P300 character speller blinks:
[0073] The P300 speller sets a total of 36 target characters, which are 26 English letters "A-Z", Arabic numerals "1-9" and short underscore "_". 36 characters are arranged in a 6×6 matrix format, and each row and...
PUM
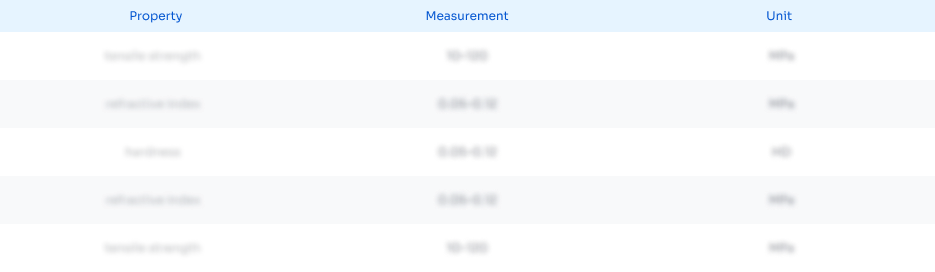
Abstract
Description
Claims
Application Information
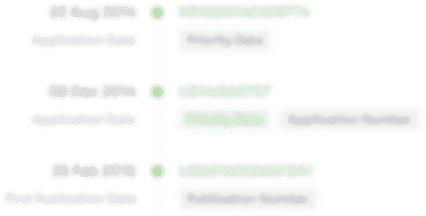
- R&D Engineer
- R&D Manager
- IP Professional
- Industry Leading Data Capabilities
- Powerful AI technology
- Patent DNA Extraction
Browse by: Latest US Patents, China's latest patents, Technical Efficacy Thesaurus, Application Domain, Technology Topic, Popular Technical Reports.
© 2024 PatSnap. All rights reserved.Legal|Privacy policy|Modern Slavery Act Transparency Statement|Sitemap|About US| Contact US: help@patsnap.com