Data dimension reduction method and device
A technology for data dimension reduction and low-dimensional data, applied in the field of data processing, can solve problems such as not being as complete as the original sample, unable to adapt to large data set dimension reduction, information loss, etc.
- Summary
- Abstract
- Description
- Claims
- Application Information
AI Technical Summary
Problems solved by technology
Method used
Image
Examples
Embodiment Construction
[0039] In order to make the purpose, technical solutions and advantages of the embodiments of the present invention more clear, the embodiments of the present invention will be further described in detail below in conjunction with the accompanying drawings. Here, the exemplary embodiments and descriptions of the present invention are used to explain the present invention, but not to limit the present invention.
[0040] figure 1 is a schematic flowchart of a data dimensionality reduction method according to an embodiment of the present invention. Such as figure 1 As shown, the data dimensionality reduction method of some embodiments may include:
[0041] Step S110: Construct an initial neural network for data dimensionality reduction, and use a high-dimensional data set and a low-dimensional data set as the input and output of the initial neural network, respectively, and the dimensionality of the sample points in the high-dimensional data set is greater than The dimensiona...
PUM
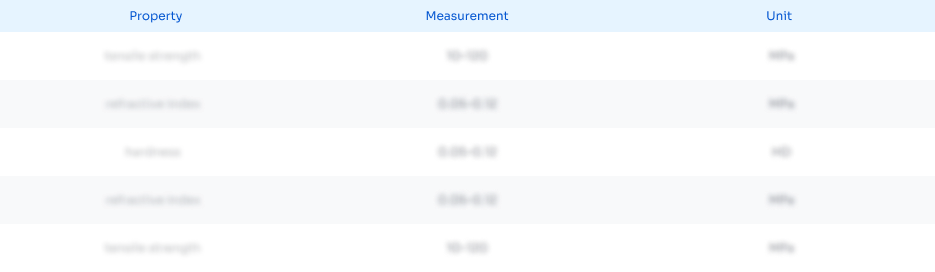
Abstract
Description
Claims
Application Information
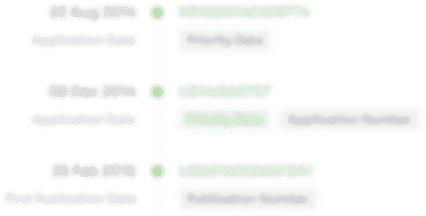
- R&D
- Intellectual Property
- Life Sciences
- Materials
- Tech Scout
- Unparalleled Data Quality
- Higher Quality Content
- 60% Fewer Hallucinations
Browse by: Latest US Patents, China's latest patents, Technical Efficacy Thesaurus, Application Domain, Technology Topic, Popular Technical Reports.
© 2025 PatSnap. All rights reserved.Legal|Privacy policy|Modern Slavery Act Transparency Statement|Sitemap|About US| Contact US: help@patsnap.com