A lung parenchyma CT image segmentation method based on a deep convolutional neural network
A neural network and deep convolution technology, applied in the field of medical image processing and deep learning, can solve the problems of difficult segmentation of lungs, unsatisfactory segmentation effect, and limited practical application, etc., to improve accuracy, fast algorithm speed, and reduce calculation volume effect
- Summary
- Abstract
- Description
- Claims
- Application Information
AI Technical Summary
Problems solved by technology
Method used
Image
Examples
Embodiment Construction
[0018] The detailed description is as follows with reference to the attached preferred embodiments, and the described preferred embodiments are only used to illustrate the technical solution of the present invention, not to limit the present invention.
[0019] figure 1 A flow chart of the lung parenchyma CT image segmentation method based on the deep convolutional neural network according to the present invention is given. Such as figure 1 Shown, according to the lung parenchyma CT image segmentation method based on deep convolutional neural network of the present invention comprises:
[0020] Step 1: collecting lung CT images;
[0021] Step 2: Use the VGG-16-based convolutional neural network improved by dilated convolution to extract multi-scale semantic information;
[0022] Step 3: Construct a multi-scale feature hypercolumn descriptor based on multi-layer extraction to extract hypercolumn features of pixels in the image;
[0023] Step 4: Learn a non-linear predictor ...
PUM
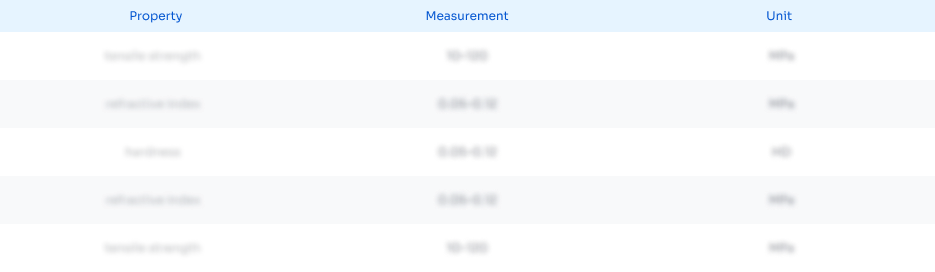
Abstract
Description
Claims
Application Information
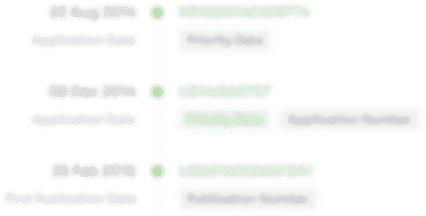
- R&D Engineer
- R&D Manager
- IP Professional
- Industry Leading Data Capabilities
- Powerful AI technology
- Patent DNA Extraction
Browse by: Latest US Patents, China's latest patents, Technical Efficacy Thesaurus, Application Domain, Technology Topic, Popular Technical Reports.
© 2024 PatSnap. All rights reserved.Legal|Privacy policy|Modern Slavery Act Transparency Statement|Sitemap|About US| Contact US: help@patsnap.com