quantum optimization parameter adjustment method for distributed deep learning under a Spark framework
A deep learning and distributed technology, applied in neural learning methods, biological models, instruments, etc., can solve problems such as lack of prior knowledge, insufficient data samples, lack of truly valuable data, etc., to avoid prior knowledge Effect
- Summary
- Abstract
- Description
- Claims
- Application Information
AI Technical Summary
Problems solved by technology
Method used
Image
Examples
Embodiment Construction
[0051] Referring to the accompanying drawings, this embodiment describes the present invention by taking cardiovascular and cerebrovascular diseases as the specific application field. The traditional deep learning model includes three links: data collection, deep learning training and model evaluation. On the basis of the above, the present invention boils down parameter tuning to finding optimal parameters, and adds a quantum optimization tuning link, such as figure 1 shown. The process flow of the quantum optimization tuning method for distributed deep learning under the Spark framework is as follows: figure 2 As shown, the detailed steps are as follows:
[0052] Step1: Collect data and perform preprocessing and grouping, which specifically includes the following four steps:
[0053] Step1.1: Extract the data of patients related to cardiovascular and cerebrovascular diseases from massive medical data, and perform distributed storage based on the distributed file system (H...
PUM
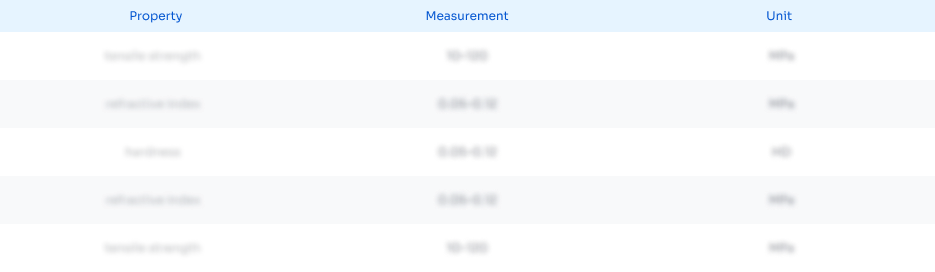
Abstract
Description
Claims
Application Information
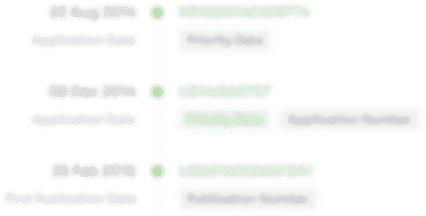
- R&D
- Intellectual Property
- Life Sciences
- Materials
- Tech Scout
- Unparalleled Data Quality
- Higher Quality Content
- 60% Fewer Hallucinations
Browse by: Latest US Patents, China's latest patents, Technical Efficacy Thesaurus, Application Domain, Technology Topic, Popular Technical Reports.
© 2025 PatSnap. All rights reserved.Legal|Privacy policy|Modern Slavery Act Transparency Statement|Sitemap|About US| Contact US: help@patsnap.com