Self-supervised learning model training method and device based on relational reasoning
A learning model and model training technology, applied in the field of computer vision and machine learning, can solve problems such as performance degradation, hindering representation migration, and large impact of representation learning, so as to achieve the effect of easy migration and reduced impact
- Summary
- Abstract
- Description
- Claims
- Application Information
AI Technical Summary
Problems solved by technology
Method used
Image
Examples
Embodiment Construction
[0029] Embodiments of the present invention are described in detail below, examples of which are shown in the drawings, wherein the same or similar reference numerals designate the same or similar elements or elements having the same or similar functions throughout. The embodiments described below by referring to the figures are exemplary and are intended to explain the present invention and should not be construed as limiting the present invention.
[0030] The method and device for training a self-supervised learning model based on relational reasoning in an embodiment of the present invention will be described below with reference to the accompanying drawings.
[0031] figure 1 It is a schematic flowchart of a self-supervised learning model training method based on relational reasoning provided by an embodiment of the present invention.
[0032] Such as figure 1 As shown, the method includes the following steps:
[0033] Step 101, acquire a set of sample images, and perf...
PUM
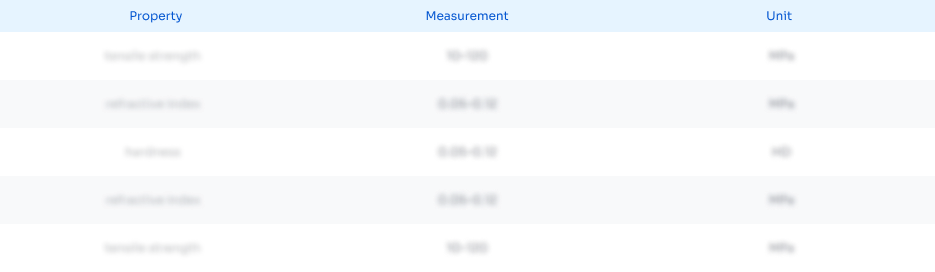
Abstract
Description
Claims
Application Information
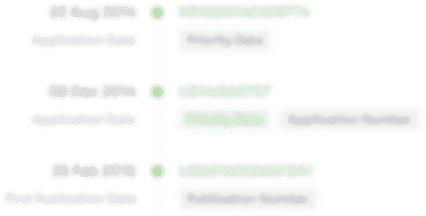
- R&D Engineer
- R&D Manager
- IP Professional
- Industry Leading Data Capabilities
- Powerful AI technology
- Patent DNA Extraction
Browse by: Latest US Patents, China's latest patents, Technical Efficacy Thesaurus, Application Domain, Technology Topic, Popular Technical Reports.
© 2024 PatSnap. All rights reserved.Legal|Privacy policy|Modern Slavery Act Transparency Statement|Sitemap|About US| Contact US: help@patsnap.com