A lung anatomy location positioning algorithm based on a deep learning technology
A deep learning and localization algorithm technology, applied in computing, image data processing, instruments, etc., can solve problems such as difficulty in extracting lung fissures, affecting the segmentation effect, and incomplete lung fissures, avoiding the risk of overfitting and ensuring generalization. Ability to ensure the effect of segmentation accuracy
- Summary
- Abstract
- Description
- Claims
- Application Information
AI Technical Summary
Problems solved by technology
Method used
Image
Examples
Embodiment Construction
[0020] The following will be combined with Figure 1-Figure 2 The present invention is described in detail, and the technical solutions in the embodiments of the present invention are clearly and completely described. Apparently, the described embodiments are only some of the embodiments of the present invention, not all of them. Based on the embodiments of the present invention, all other embodiments obtained by persons of ordinary skill in the art without making creative efforts belong to the protection scope of the present invention.
[0021] The present invention provides a lung anatomical position positioning algorithm based on deep learning technology through improvement; this patent proposes an end-to-end deep learning network, which can accurately and quickly divide lung CT, and the process is simple, roughly as follows :
[0022] (1) Data type conversion is performed on the input CT, and the pixel value of the image is converted from a 32-bit floating-point number ty...
PUM
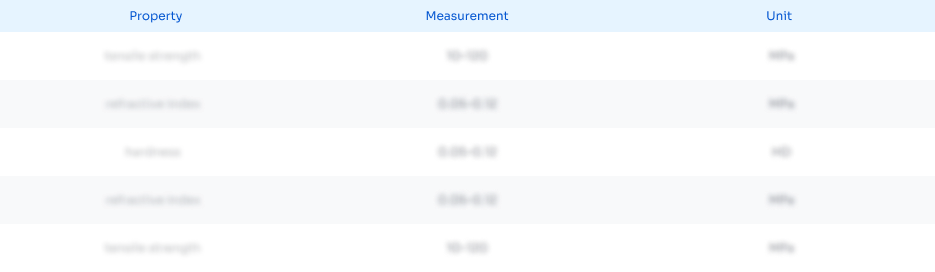
Abstract
Description
Claims
Application Information
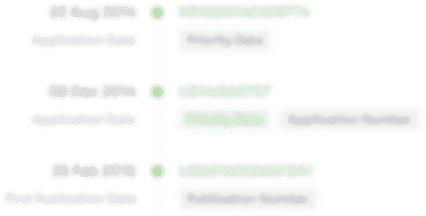
- R&D Engineer
- R&D Manager
- IP Professional
- Industry Leading Data Capabilities
- Powerful AI technology
- Patent DNA Extraction
Browse by: Latest US Patents, China's latest patents, Technical Efficacy Thesaurus, Application Domain, Technology Topic, Popular Technical Reports.
© 2024 PatSnap. All rights reserved.Legal|Privacy policy|Modern Slavery Act Transparency Statement|Sitemap|About US| Contact US: help@patsnap.com