A deep reinforcement learning method and equipment based on a plurality of historical optimal Q networks
A reinforcement learning and network technology, applied in the field of deep reinforcement learning, can solve problems such as low interactive evaluation scores, and achieve the effects of reducing the amount of calculation, stabilizing the training process, and improving the efficiency of use.
- Summary
- Abstract
- Description
- Claims
- Application Information
AI Technical Summary
Problems solved by technology
Method used
Image
Examples
Embodiment Construction
[0048] In the following, specific embodiments of the present invention will be described in detail in conjunction with the examples and accompanying drawings. The embodiments depicted here are only used to illustrate and explain the present invention, but not to limit the present invention.
[0049] Aiming at the interactive system of intelligent robots including agents, the deep reinforcement learning method based on multiple historical best Q-networks proposed by the present invention, such as figure 1 As shown, it mainly includes the following steps: First, define the attributes and rules of the single agent, clarify the state space and action space of the agent, construct or call the single-agent motion interaction environment, that is, observe the environment o, according to the strategy π, the intelligence Then, based on the interactive evaluation score, select the best Q-networks from all historical Q-networks according to the interactive evaluation score of each round;...
PUM
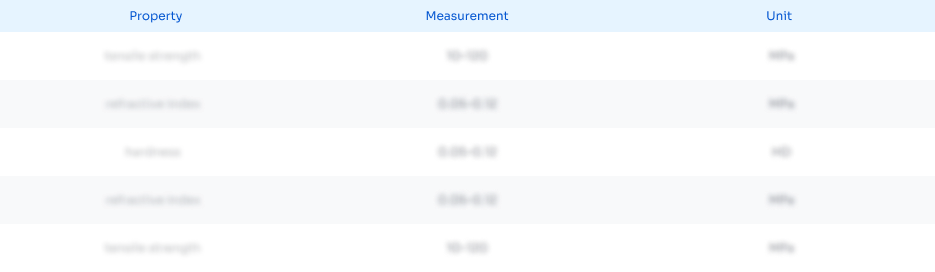
Abstract
Description
Claims
Application Information
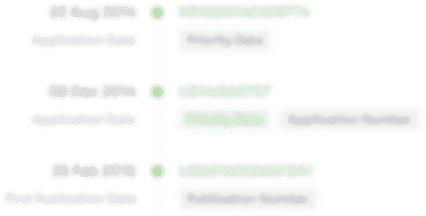
- R&D Engineer
- R&D Manager
- IP Professional
- Industry Leading Data Capabilities
- Powerful AI technology
- Patent DNA Extraction
Browse by: Latest US Patents, China's latest patents, Technical Efficacy Thesaurus, Application Domain, Technology Topic, Popular Technical Reports.
© 2024 PatSnap. All rights reserved.Legal|Privacy policy|Modern Slavery Act Transparency Statement|Sitemap|About US| Contact US: help@patsnap.com