Intelligent network connection vehicle preceding vehicle acceleration prediction method based on Gaussian process regression
A technology of Gaussian process regression and prediction method, which is applied in the field of ahead vehicle acceleration prediction of intelligent networked vehicles based on Gaussian process regression, which can solve the problems of difficulty in predicting the acceleration of the preceding vehicle, and difficulty in predicting the acceleration of the preceding vehicle.
- Summary
- Abstract
- Description
- Claims
- Application Information
AI Technical Summary
Problems solved by technology
Method used
Image
Examples
Embodiment Construction
[0043] The method of the present invention will be described in further detail below in conjunction with the accompanying drawings.
[0044] refer to figure 1 and figure 2 , a method for predicting the acceleration of a front vehicle based on a Gaussian process regression, the method comprising the steps of:
[0045] 1) Select the training set sample, and measure the acceleration data a(t-10), a(t-9),...,a(t-1) of the vehicle in front at 10 (n takes 10) historical moments at time t, so that time x i =t-11+i, acceleration sample value y i =a(t-11+i), i=1,2,...,10, let X={x 1 ,x 2 ,...,x i} is expressed as 10 historical moments at the current moment t, Y={y 1 ,y 2 ,...,y i} is expressed as the acceleration data of the vehicle in front at 10 historical moments measured at the current moment t; X is used as the input of the training set, and Y is used as the output of the training set, where Y obeys the Gaussian distribution, see formula (1):
[0046] Y=F(X,X)~N(M(X,X),K(...
PUM
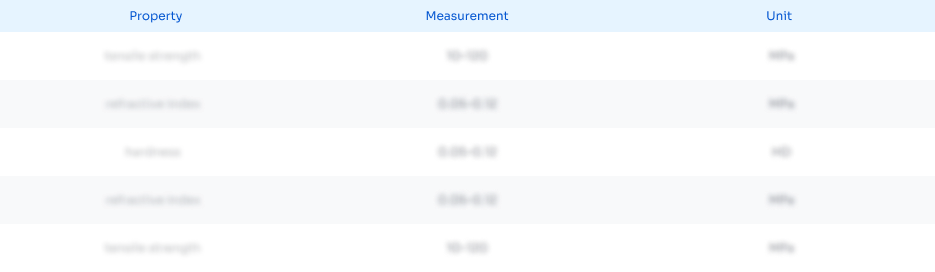
Abstract
Description
Claims
Application Information
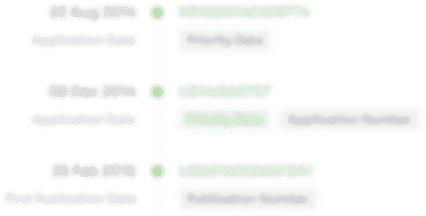
- Generate Ideas
- Intellectual Property
- Life Sciences
- Materials
- Tech Scout
- Unparalleled Data Quality
- Higher Quality Content
- 60% Fewer Hallucinations
Browse by: Latest US Patents, China's latest patents, Technical Efficacy Thesaurus, Application Domain, Technology Topic, Popular Technical Reports.
© 2025 PatSnap. All rights reserved.Legal|Privacy policy|Modern Slavery Act Transparency Statement|Sitemap|About US| Contact US: help@patsnap.com