Convolutional neural network road scene classification and road segmentation method
A convolutional neural network and scene classification technology, which is applied in the field of convolutional neural network road scene classification and road segmentation to achieve accurate segmentation, effective and reliable driving assistance functions, and improve the functions of intelligent driving assistance systems.
- Summary
- Abstract
- Description
- Claims
- Application Information
AI Technical Summary
Problems solved by technology
Method used
Image
Examples
Embodiment Construction
[0028] 1. System composition
[0029] The present invention realizes road scene classification and road area segmentation and is divided into two parts. The first part is a training part, and network training is performed first. This is done offline before the system is used. The second part is the test part, which uses the trained network to discriminate the input road image. The processing steps of the two parts are the same, and the specific steps are described later (3. Processing flow). The difference between the two parts is that the input data is different. The input data of the first part is the training data set prepared in advance as needed. The input data for the second part is the test dataset. The test dataset can be pre-prepared untrained images. The training data and test data can also be composed of real-time road images collected in the field.
[0030] 2. Dataset
[0031] The data set used in the present invention is composed of 4000 road scene images i...
PUM
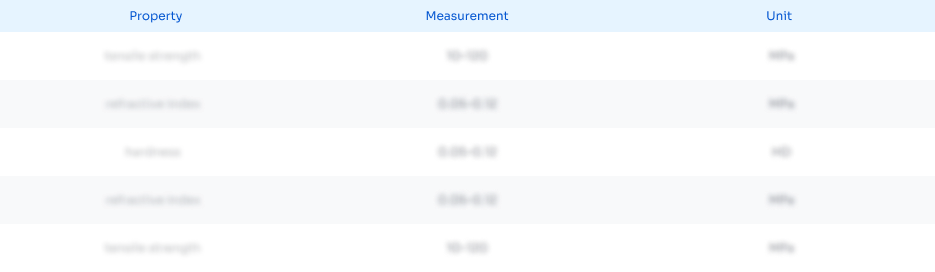
Abstract
Description
Claims
Application Information
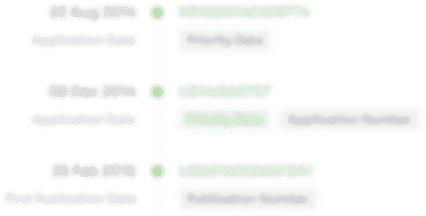
- Generate Ideas
- Intellectual Property
- Life Sciences
- Materials
- Tech Scout
- Unparalleled Data Quality
- Higher Quality Content
- 60% Fewer Hallucinations
Browse by: Latest US Patents, China's latest patents, Technical Efficacy Thesaurus, Application Domain, Technology Topic, Popular Technical Reports.
© 2025 PatSnap. All rights reserved.Legal|Privacy policy|Modern Slavery Act Transparency Statement|Sitemap|About US| Contact US: help@patsnap.com