Convolutional neural network optimization and rapid target detection method and device
A convolutional neural network and optimization method technology, applied in the field of convolutional neural network optimization and fast target detection, can solve problems such as slow running speed and large volume of convolutional neural network models, to improve speed, reduce memory or The effect of memory space and improving calculation speed
- Summary
- Abstract
- Description
- Claims
- Application Information
AI Technical Summary
Benefits of technology
Problems solved by technology
Method used
Image
Examples
Embodiment Construction
[0027] In order to make the purpose, technical solutions and advantages of the embodiments of the present invention clearer, the technical solutions in the embodiments of the present invention will be clearly and completely described below in conjunction with the drawings in the embodiments of the present invention. Obviously, the described embodiments It is a part of embodiments of the present invention, but not all embodiments. Based on the embodiments of the present invention, all other embodiments obtained by those skilled in the art without creative efforts fall within the protection scope of the present invention.
[0028] An artificial neural network is a network composed of multiple interconnected neurons. like figure 1 As shown, the white circle in the figure represents a neuron. Each neuron is composed of a weight value, a bias, and an activation function. The neuron converts the input data through a linear transformation based on the weight value and offset; the ac...
PUM
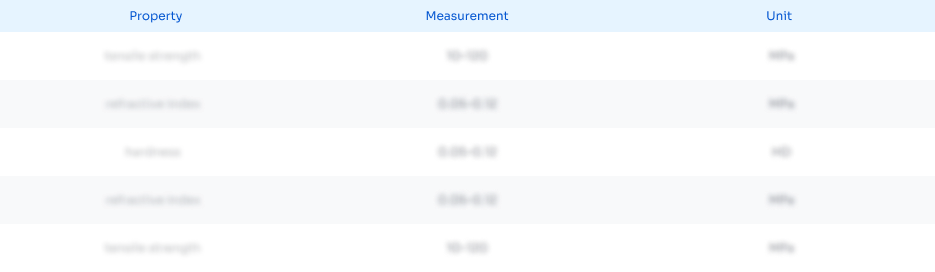
Abstract
Description
Claims
Application Information
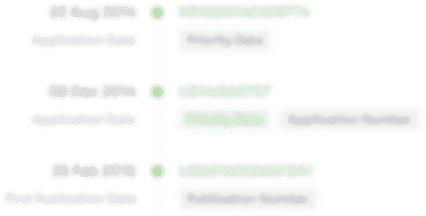
- R&D Engineer
- R&D Manager
- IP Professional
- Industry Leading Data Capabilities
- Powerful AI technology
- Patent DNA Extraction
Browse by: Latest US Patents, China's latest patents, Technical Efficacy Thesaurus, Application Domain, Technology Topic, Popular Technical Reports.
© 2024 PatSnap. All rights reserved.Legal|Privacy policy|Modern Slavery Act Transparency Statement|Sitemap|About US| Contact US: help@patsnap.com