Deep learning semantic segmentation model compression method for embedded mobile terminal
A technology of deep learning and semantic segmentation, applied to biological neural network models, character and pattern recognition, instruments, etc., can solve the problems of graphics processors with weak heat dissipation capabilities, affect model operation time, consume calculation time, etc., and achieve faster forward The effect of guessing speed, reducing the amount of model parameters, and fast semantic segmentation
- Summary
- Abstract
- Description
- Claims
- Application Information
AI Technical Summary
Problems solved by technology
Method used
Image
Examples
Embodiment Construction
[0046] The present invention will be further described below in conjunction with the accompanying drawings.
[0047] combine figure 1 , a deep learning semantic segmentation model compression method for embedded mobile platforms. The main distillation framework includes a teacher network, a student network, a discrimination network, and three different levels of distillation structures (pairwise distillation, pixel distillation, and overall distillation), Three kinds of loss functions inside the network (discrimination loss function, segmentation loss function, distillation loss function), where:
[0048]The teacher network prototype is based on the deep learning ESPNet segmentation network model, receives the input original image, outputs the classification probability value and classification result of each class on the feature map and corresponding pixels, and sends it to the loss function for the next step.
[0049] The student network is a small network that needs to be ...
PUM
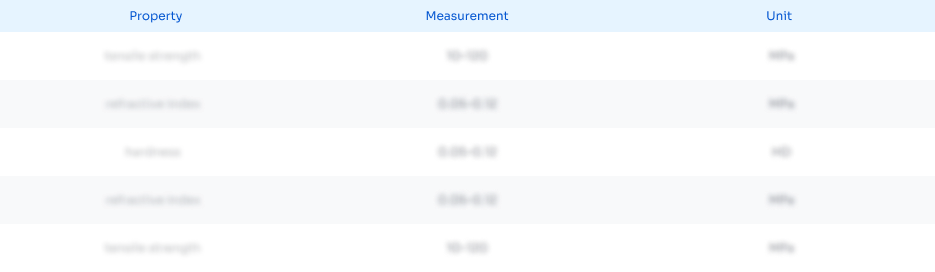
Abstract
Description
Claims
Application Information
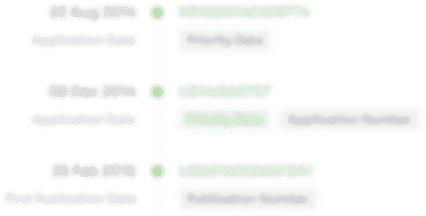
- R&D Engineer
- R&D Manager
- IP Professional
- Industry Leading Data Capabilities
- Powerful AI technology
- Patent DNA Extraction
Browse by: Latest US Patents, China's latest patents, Technical Efficacy Thesaurus, Application Domain, Technology Topic, Popular Technical Reports.
© 2024 PatSnap. All rights reserved.Legal|Privacy policy|Modern Slavery Act Transparency Statement|Sitemap|About US| Contact US: help@patsnap.com