A single image 3D object reconstruction method based on deep learning
A single image, deep learning technology, applied in image data processing, 3D image processing, instruments, etc., can solve the problems of limited use, algorithm complexity and immature hardware, depth camera, etc., to achieve the effect of good understanding ability
- Summary
- Abstract
- Description
- Claims
- Application Information
AI Technical Summary
Problems solved by technology
Method used
Image
Examples
Embodiment
[0029] figure 1 It is a flowchart of a method for dynamic three-dimensional reconstruction of a human body according to an embodiment of the present invention. figure 1 , detailing each step.
[0030] Step S110, inputting a single-color image containing multiple objects.
[0031] A single image is taken with a normal camera and contains RGB colormaps of one or more objects of the same class. The limitation of "same category" corresponds to the shape space in the subsequent sampling sub-network. In the implementation process, the application scenario should be determined first, that is, the class to which the rigid body object belongs to be reconstructed, and then the shape sampling network uses the transfer learning method. The shape space sampler corresponding to this type of object can be obtained by simply iterating the existing weights of the point cloud model of this type in the pre-trained class. In addition, the input image can also be an RGBD image, and the method i...
PUM
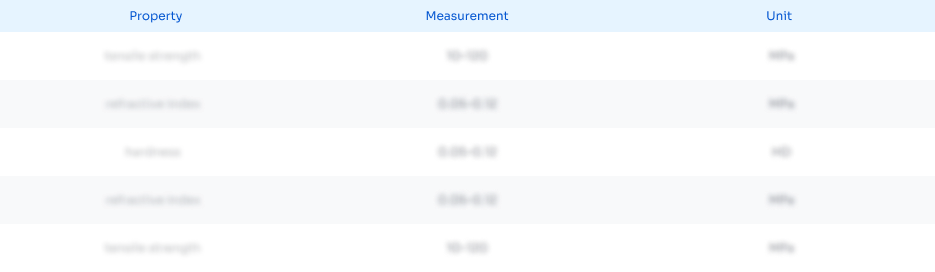
Abstract
Description
Claims
Application Information
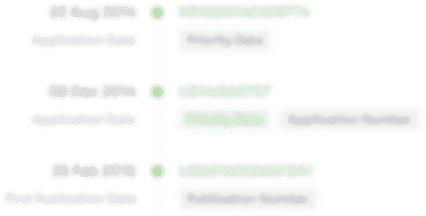
- Generate Ideas
- Intellectual Property
- Life Sciences
- Materials
- Tech Scout
- Unparalleled Data Quality
- Higher Quality Content
- 60% Fewer Hallucinations
Browse by: Latest US Patents, China's latest patents, Technical Efficacy Thesaurus, Application Domain, Technology Topic, Popular Technical Reports.
© 2025 PatSnap. All rights reserved.Legal|Privacy policy|Modern Slavery Act Transparency Statement|Sitemap|About US| Contact US: help@patsnap.com