Semi-supervised image classification method based on generative adversarial network
A classification method and network technology, applied in the field of image processing, can solve the problems of not meeting the requirements of classification, lack of training labels, and limited application range, etc., and achieve the effect of accurate image classification accuracy, wide applicability, and improved accuracy
- Summary
- Abstract
- Description
- Claims
- Application Information
AI Technical Summary
Problems solved by technology
Method used
Image
Examples
Embodiment Construction
[0027] The embodiments and effects of the present invention will be further described in detail below in conjunction with the accompanying drawings.
[0028] refer to figure 1 , the concrete steps of the present invention are as follows.
[0029] Step 1. Select and download the image classification standard training sample set.
[0030] Download the standard image classification data set mnist handwritten data set, and normalize the data samples for network model training;
[0031] Download the cifar10 dataset, a standard image classification dataset, and normalize the data samples for network model training.
[0032] Step 2, setting supervised learning parameters.
[0033] Count the number of training samples in the training sample set, and control the percentage of supervised learning label data in the total number of training samples. The higher the percentage of label data in the total number of training samples, the better the model training accuracy, but it is difficu...
PUM
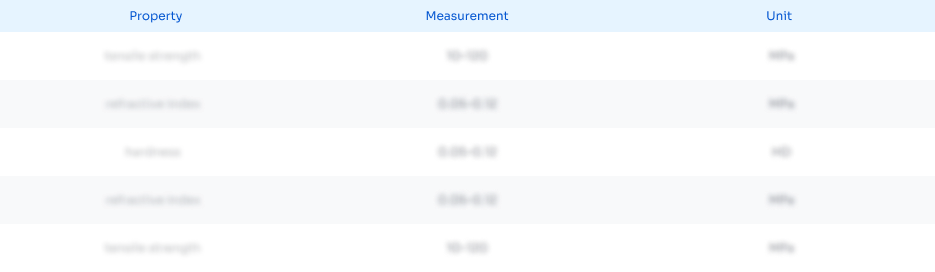
Abstract
Description
Claims
Application Information
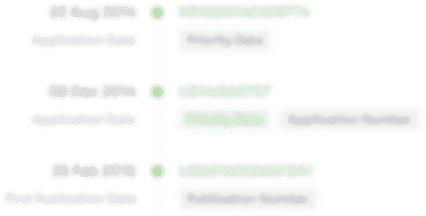
- R&D Engineer
- R&D Manager
- IP Professional
- Industry Leading Data Capabilities
- Powerful AI technology
- Patent DNA Extraction
Browse by: Latest US Patents, China's latest patents, Technical Efficacy Thesaurus, Application Domain, Technology Topic, Popular Technical Reports.
© 2024 PatSnap. All rights reserved.Legal|Privacy policy|Modern Slavery Act Transparency Statement|Sitemap|About US| Contact US: help@patsnap.com