Semi-offline deep target tracking method based on deep learning
A target tracking and deep learning technology, applied in the field of semi-offline deep target tracking based on deep learning, can solve problems such as target recognition performance decline and target recognition failure, so as to reduce the probability of recognition failure, ensure recognition accuracy, and improve speed. Effect
- Summary
- Abstract
- Description
- Claims
- Application Information
AI Technical Summary
Problems solved by technology
Method used
Image
Examples
Embodiment Construction
[0024] Such as figure 1 As shown, the present invention is a semi-offline depth target tracking method based on deep learning, which includes the following steps.
[0025] S1: build the tracker network model, build the structure of the shared layer of the tracker network model based on MDNet;
[0026] The tracker network model of the semi-offline deep target tracker based on deep learning includes a shared layer and a specific domain layer; The weight of the domain layer allows it to adapt to specific target features, so as to achieve the purpose of identifying the target and background from the collected samples, and realize the determination of the target position.
[0027] The shared layer includes 3 consecutive convolutional layers and 2 consecutive fully connected layers connected in sequence; the network model receives pictures to be classified through the input layer, these pictures are pictures with 3 channels and a size of 107×107, and the input layer receives The p...
PUM
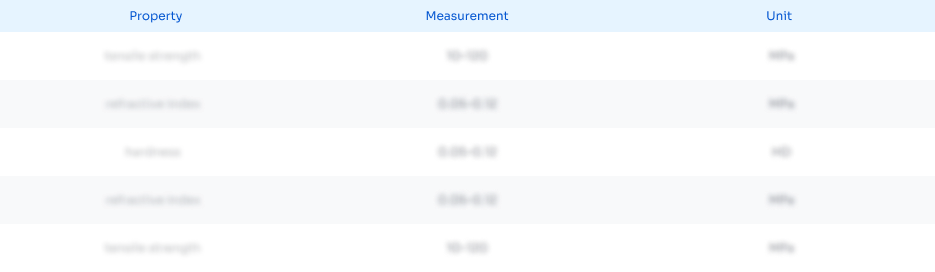
Abstract
Description
Claims
Application Information
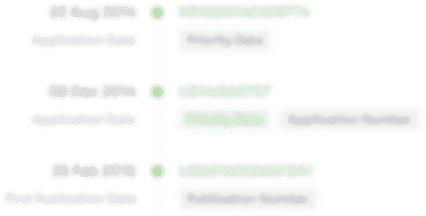
- R&D
- Intellectual Property
- Life Sciences
- Materials
- Tech Scout
- Unparalleled Data Quality
- Higher Quality Content
- 60% Fewer Hallucinations
Browse by: Latest US Patents, China's latest patents, Technical Efficacy Thesaurus, Application Domain, Technology Topic, Popular Technical Reports.
© 2025 PatSnap. All rights reserved.Legal|Privacy policy|Modern Slavery Act Transparency Statement|Sitemap|About US| Contact US: help@patsnap.com