Adaptive adversarial learning-based urban traffic scene semantic segmentation method and system
A technology of semantic segmentation and urban transportation, applied in neural learning methods, image analysis, image data processing, etc., can solve the problems of affecting segmentation accuracy, low segmentation accuracy, complex scenes, etc., to achieve the effect of enhancing generalization ability
- Summary
- Abstract
- Description
- Claims
- Application Information
AI Technical Summary
Problems solved by technology
Method used
Image
Examples
Embodiment Construction
[0055] The following will clearly and completely describe the technical solutions in the embodiments of the present invention with reference to the accompanying drawings in the embodiments of the present invention. Obviously, the described embodiments are only some, not all, embodiments of the present invention. Based on the embodiments of the present invention, all other embodiments obtained by persons of ordinary skill in the art without making creative efforts belong to the protection scope of the present invention.
[0056] The purpose of the present invention is to provide a method and system for semantic segmentation of urban traffic scenes based on self-adaptive adversarial learning, improve the semantic segmentation accuracy of complex urban traffic scenes lacking labeling information and with multi-scale targets, and enhance the generalization ability of semantic segmentation models.
[0057] In order to make the above objects, features and advantages of the present in...
PUM
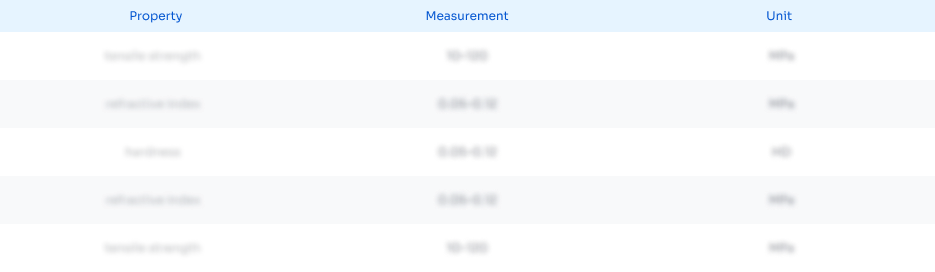
Abstract
Description
Claims
Application Information
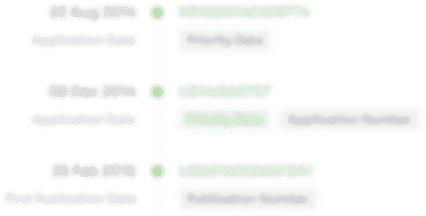
- R&D
- Intellectual Property
- Life Sciences
- Materials
- Tech Scout
- Unparalleled Data Quality
- Higher Quality Content
- 60% Fewer Hallucinations
Browse by: Latest US Patents, China's latest patents, Technical Efficacy Thesaurus, Application Domain, Technology Topic, Popular Technical Reports.
© 2025 PatSnap. All rights reserved.Legal|Privacy policy|Modern Slavery Act Transparency Statement|Sitemap|About US| Contact US: help@patsnap.com