A Fast Multi-Scale Estimation Object Tracking Method on Re-Detection
A re-detection and target tracking technology, which is applied in the fields of image processing and computer vision, can solve problems such as error and slow tracking speed, and achieve the effect of solving fast motion, improving computing speed, and improving feature expression ability
- Summary
- Abstract
- Description
- Claims
- Application Information
AI Technical Summary
Problems solved by technology
Method used
Image
Examples
Embodiment 1
[0069] The system flow frame diagram of re-detected target tracking is as follows figure 1 As shown, it includes the following parts: training filter template, localization, detection, re-detection, and model update.
[0070] (1) Step 1: Train the filter template. First, initialize the target tracker, mark the initial area of the target, use the VGG-19 network to extract the depth features of the target, and establish the initial target template and scale template for the calculation of the target response value in the second frame.
[0071] The establishment of the target template is mainly divided into the following parts:
[0072] First, the classifier performs cyclic shift sampling on an image block of size M×N with the target position as the center, and the resulting sample set is denoted as x i , where i∈{0,…M-1}×{0,…N-1}. each sample x i has a corresponding regression label y i , y i obtained from the Gaussian function. The purpose of the classifier f(x) is to ...
example
[0119] The invention measures the performance of the tracking algorithm through the OPE (one pass evaluation) evaluation standard, and selects 60 challenging video sequences from the OTB100 data set for analysis, and compares it with other trackers (DeepKCF, SAMF, KCF, CSK, DFT) , CT, CACF and other 7 trackers) are compared under different challenge factors (illumination change, target deformation, motion blur, fast motion, in-plane rotation, out-of-plane rotation, target out of field of view, background clutter, low resolution, etc.) .
[0120] Figure 4 are the sampling frames of the tracking results of the tracking method of the present invention (DRKCF) and other seven trackers, from Figure 4 It can be seen that the tracker proposed by the present invention can track the target better than other trackers, and even if the target is lost, the target can be retrieved to continue tracking.
[0121] Figure 5 is the comparison of the tracking method of the present inventi...
PUM
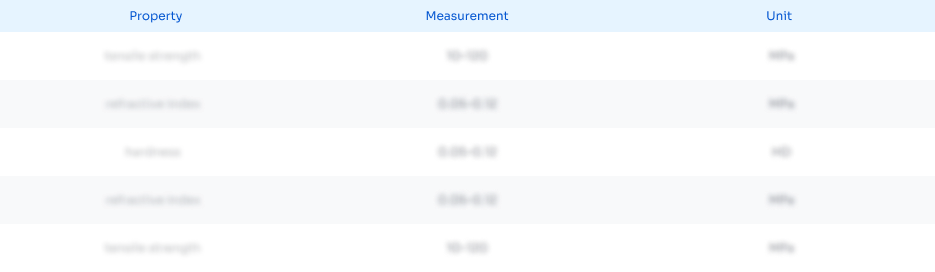
Abstract
Description
Claims
Application Information
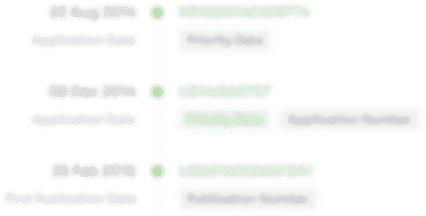
- R&D Engineer
- R&D Manager
- IP Professional
- Industry Leading Data Capabilities
- Powerful AI technology
- Patent DNA Extraction
Browse by: Latest US Patents, China's latest patents, Technical Efficacy Thesaurus, Application Domain, Technology Topic, Popular Technical Reports.
© 2024 PatSnap. All rights reserved.Legal|Privacy policy|Modern Slavery Act Transparency Statement|Sitemap|About US| Contact US: help@patsnap.com