Traffic flow prediction method based on deep neural network integration
A deep neural network and traffic flow technology, applied in the field of traffic flow prediction based on deep neural network integration, can solve problems such as poor prediction performance, effective information affecting model performance, and inability to handle the nonlinear characteristics of traffic flow data well.
- Summary
- Abstract
- Description
- Claims
- Application Information
AI Technical Summary
Problems solved by technology
Method used
Image
Examples
Embodiment 1
[0089] Embodiment 1 uses a test set to test the predictive performance of the present invention.
[0090] Example 1
[0091] In the following, the prediction performance of the present invention is illustrated through experiments on the PeMS traffic flow data set, and the performance is compared with the SARIMA (seasonal sum autoregressive moving average) model. On the PeMS data set, the traffic flow data of an observation site on all working days from January 1, 2018 to September 30, 2018 was selected as the experimental data set, and a total of 4536 measurement data were obtained. Among them, the first 4036 data are taken to form the training set, and the last 500 data are used to form the test set, and all data are normalized by maximum and minimum values.
[0092] For the SARIMA prediction model, the model is constructed on the training set according to the Box-Jenkins analysis method, and finally a more suitable prediction model SARIMA(8,1,2)(0,1,1) is obtained 24 , the...
PUM
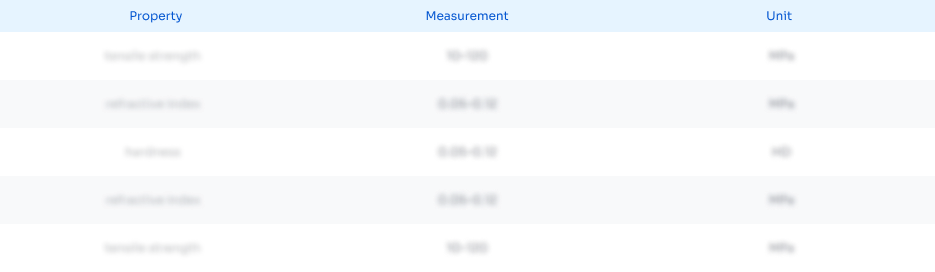
Abstract
Description
Claims
Application Information
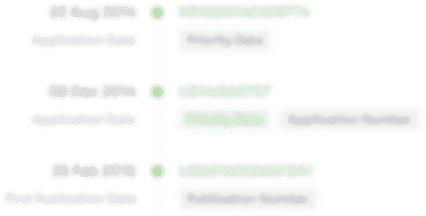
- R&D Engineer
- R&D Manager
- IP Professional
- Industry Leading Data Capabilities
- Powerful AI technology
- Patent DNA Extraction
Browse by: Latest US Patents, China's latest patents, Technical Efficacy Thesaurus, Application Domain, Technology Topic.
© 2024 PatSnap. All rights reserved.Legal|Privacy policy|Modern Slavery Act Transparency Statement|Sitemap