Super-resolution reconstruction method based on dense neural network and two-parameter loss function
A technology of super-resolution reconstruction and loss function, which is applied in the field of image processing, can solve the problem of not being able to reconstruct high-frequency information images, etc., achieve good generalization ability, save pre-processing and post-processing, and increase the effect of reuse
- Summary
- Abstract
- Description
- Claims
- Application Information
AI Technical Summary
Problems solved by technology
Method used
Image
Examples
Embodiment
[0058] 1. Data preparation:
[0059] (a) Divide the dataset. The public dataset DIV2K is used, which contains 800 training images, 100 verification images and 100 test images. Since the high-resolution images of the test images are not publicly available, 100 validation images are used to test the reconstruction effect. Among them, the 800 training images used for training and the 100 verification images used for testing are composed of high-resolution images and their corresponding low-resolution images. generated by the degraded model.
[0060] (b) Divide 800 training images without overlapping into 96×96 image blocks as network input.
[0061] 2. Network structure construction:
[0062] The network structure of the present invention is mainly composed of N multi-connection structural blocks, an amplification module and a jump layer. The following will combine figure 1 , and describe in detail the network structure built by the present invention. figure 1 "C" in the m...
PUM
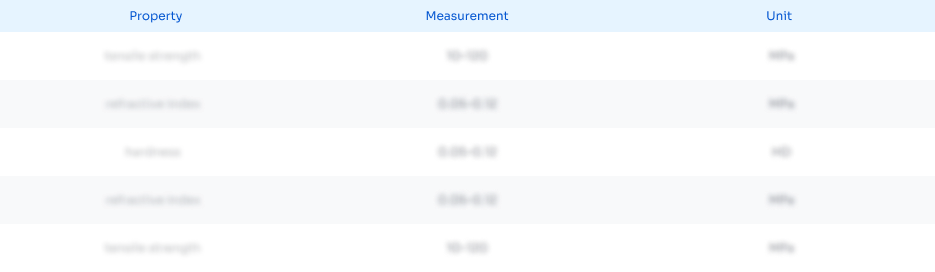
Abstract
Description
Claims
Application Information
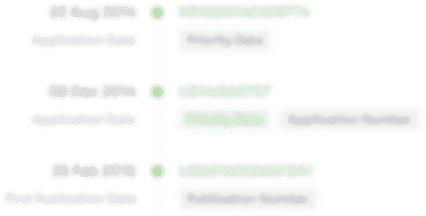
- R&D Engineer
- R&D Manager
- IP Professional
- Industry Leading Data Capabilities
- Powerful AI technology
- Patent DNA Extraction
Browse by: Latest US Patents, China's latest patents, Technical Efficacy Thesaurus, Application Domain, Technology Topic, Popular Technical Reports.
© 2024 PatSnap. All rights reserved.Legal|Privacy policy|Modern Slavery Act Transparency Statement|Sitemap|About US| Contact US: help@patsnap.com