An open-loop identification method for industrial process multi-order inertial systems based on deep learning
A deep learning and industrial process technology, applied in machine learning, character and pattern recognition, instruments, etc., can solve the problems of complex excitation signals and low accuracy of object models, and achieve the effect of simple excitation signals
- Summary
- Abstract
- Description
- Claims
- Application Information
AI Technical Summary
Problems solved by technology
Method used
Image
Examples
Embodiment Construction
[0018] In order to have a clearer understanding of the technical features, objects and effects of the present invention, the specific embodiments of the present invention will now be described with reference to the accompanying drawings.
[0019] 1. An open-loop identification method for industrial process multi-order inertial systems based on deep learning
[0020] Based on a class of multi-order inertial systems, the present invention proposes an open-loop identification method based on deep learning, which can easily and accurately identify the open-loop object model of the inertial system. The identification method proposed by the present invention is based on the deep learning random deactivation neural network, that is, the DNN network, and the first-order inertial time constant of 30 seconds and 60 seconds and the inertial time constant of 30 seconds, 60 seconds and 60 seconds are set at the input of the network. After 160 seconds of second-order inertial filtering, an ...
PUM
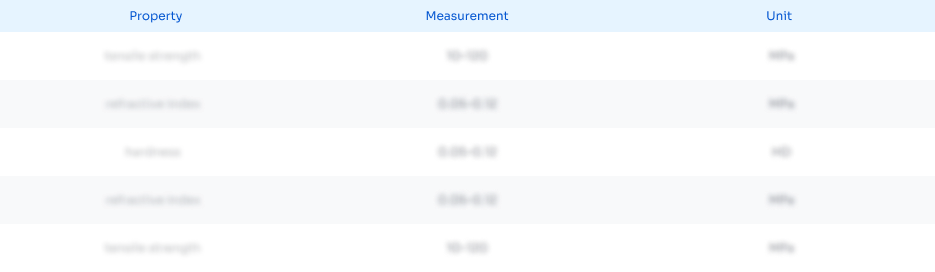
Abstract
Description
Claims
Application Information
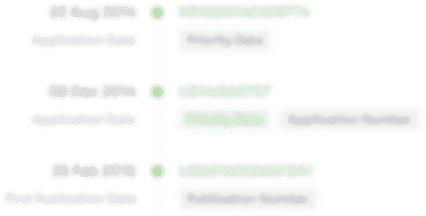
- R&D
- Intellectual Property
- Life Sciences
- Materials
- Tech Scout
- Unparalleled Data Quality
- Higher Quality Content
- 60% Fewer Hallucinations
Browse by: Latest US Patents, China's latest patents, Technical Efficacy Thesaurus, Application Domain, Technology Topic, Popular Technical Reports.
© 2025 PatSnap. All rights reserved.Legal|Privacy policy|Modern Slavery Act Transparency Statement|Sitemap|About US| Contact US: help@patsnap.com