Image question and answer method based on multi-objective association deep reasoningmulti-target association deep reasoning
A multi-target and image technology, applied in the field of deep neural network structure, can solve problems such as high degree of freedom and complex image content
- Summary
- Abstract
- Description
- Claims
- Application Information
AI Technical Summary
Problems solved by technology
Method used
Image
Examples
Embodiment Construction
[0064] The detailed parameters of the present invention will be further specifically described below.
[0065] Such as figure 1 As shown, the present invention provides a deep neural network framework for Visual Question Answering.
[0066] The data preprocessing described in step (1) and feature extraction are carried out to image and text, specifically as follows:
[0067] 1-1. For the feature extraction of image data, we use the MS-COCO dataset as training and testing data, and use the existing Faster-RCNN model to extract its visual features. Specifically, we input the image data into the Faster-RCNN network, use the Faster-RCNN model to detect and frame 10 to 100 targets in the image, extract 2048-dimensional visual features V from each target image, and record each icon The coordinates and size {x, y, w, h} of the box are used as the geometric features G of the target, where V={v 1 , v 2 ,...,v k}, G={g 1 , g 2 ,..., g k}, k ∈ [10,100].
[0068] 1-2. For the qu...
PUM
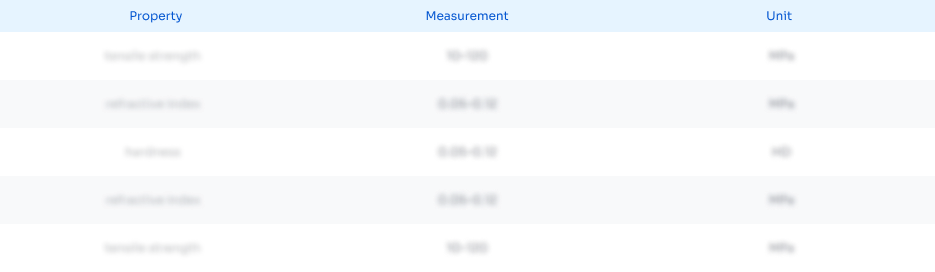
Abstract
Description
Claims
Application Information
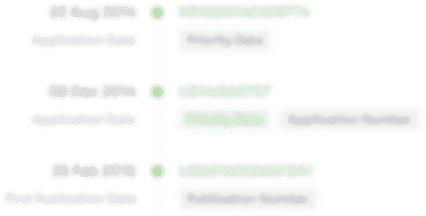
- R&D
- Intellectual Property
- Life Sciences
- Materials
- Tech Scout
- Unparalleled Data Quality
- Higher Quality Content
- 60% Fewer Hallucinations
Browse by: Latest US Patents, China's latest patents, Technical Efficacy Thesaurus, Application Domain, Technology Topic, Popular Technical Reports.
© 2025 PatSnap. All rights reserved.Legal|Privacy policy|Modern Slavery Act Transparency Statement|Sitemap|About US| Contact US: help@patsnap.com