Subjective question scoring model and scoring method based on deep learning BERT-CNN
A BERT--CNN, deep learning technology, applied in the subjective question scoring model and scoring based on deep learning BERT--CNN, subjective question scoring model and scoring field, can solve problems such as unreasonable and wasteful scoring structures, and achieve reduction Labor costs, improved performance, and the effects of overcoming inaccurate scoring results
- Summary
- Abstract
- Description
- Claims
- Application Information
AI Technical Summary
Problems solved by technology
Method used
Image
Examples
Embodiment 1
[0026] Embodiment 1: This subjective question scoring model based on deep learning BERT--CNN includes BERT conversion word vector matrix module, CNN semantic feature extraction module, similarity calculation module, scoring module, wherein BERT conversion word vector matrix module, for Convert the answer text into a word vector matrix, then pass the word vector matrix of each answer to the CNN semantic feature extraction module, and the CNN semantic feature extraction module is used to obtain the semantic feature vector of the answer text, including convolutional layers and pooling layer to obtain the semantic feature vector of each answer text, and send it to the similarity calculation module to calculate the similarity value between the semantic feature vectors of each answer text, and then send the similarity value to the scoring module to determine the answer The score of the text.
[0027] Using this deep learning BERT--CNN subjective question scoring model to score, firs...
Embodiment 2
[0036] Embodiment 2: The method in this embodiment is the same as that in Embodiment 1, except that the pooling algorithms in step (1) and step (2) are both minimum pooling methods.
Embodiment 3
[0037] Embodiment 3: The method of this embodiment is the same as that of Embodiment 1, except that the pooling algorithms in step (1) and step (2) are both average pooling methods.
PUM
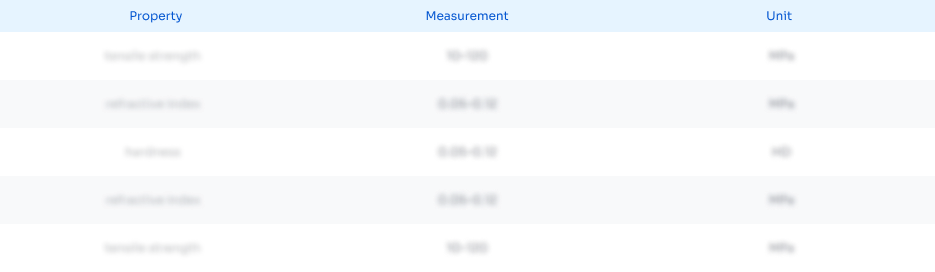
Abstract
Description
Claims
Application Information
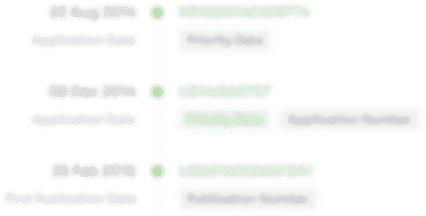
- R&D Engineer
- R&D Manager
- IP Professional
- Industry Leading Data Capabilities
- Powerful AI technology
- Patent DNA Extraction
Browse by: Latest US Patents, China's latest patents, Technical Efficacy Thesaurus, Application Domain, Technology Topic, Popular Technical Reports.
© 2024 PatSnap. All rights reserved.Legal|Privacy policy|Modern Slavery Act Transparency Statement|Sitemap|About US| Contact US: help@patsnap.com