Diaphragm flaw detection method based on reverse bottleneck structure deep convolutional network
A deep convolution and point detection technology, which is applied in the field of deep learning and computer vision, can solve the problems of low recognition accuracy and slow speed, and achieve the effects of improving accuracy, reducing the amount of parameters, and reducing costs
- Summary
- Abstract
- Description
- Claims
- Application Information
AI Technical Summary
Problems solved by technology
Method used
Image
Examples
Embodiment
[0052] Such as figure 1 As shown, a membrane defect detection method based on the reverse bottleneck structure deep convolutional network.
[0053] Step 1. Prepare the dataset
[0054] The images of the diaphragm with defects are collected from the production workshop to make a data set, which contains several pictures taken by industrial-grade cameras.
[0055] Step 2: Segment the collected defective diaphragm images into a series of small images.
[0056] Since the collected initial image is very large, and the defect points in it are very small, if the large image is directly input into the network for training, it will not only increase the difficulty of training and detection, but also cause a waste of computing resources, and it is difficult to analyze the data. Sets are labeled. Therefore, pixel-level cropping is performed on the original image, and each original image can be cropped into 800 small images of 224×224 pixels.
[0057] Step 3: Judging and labeling the ...
PUM
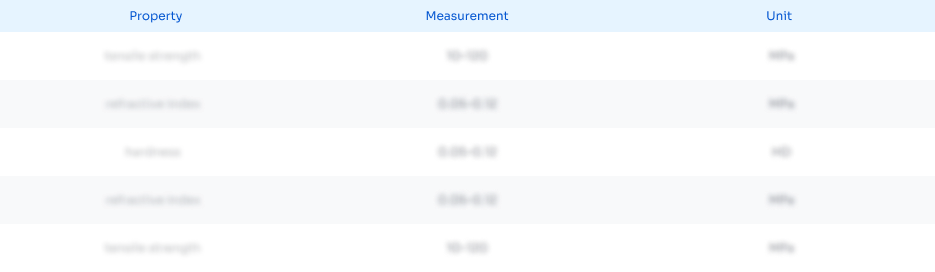
Abstract
Description
Claims
Application Information
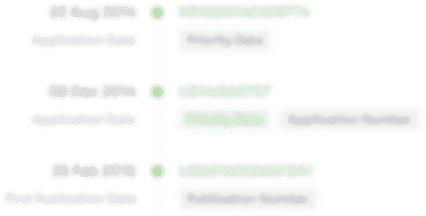
- R&D Engineer
- R&D Manager
- IP Professional
- Industry Leading Data Capabilities
- Powerful AI technology
- Patent DNA Extraction
Browse by: Latest US Patents, China's latest patents, Technical Efficacy Thesaurus, Application Domain, Technology Topic.
© 2024 PatSnap. All rights reserved.Legal|Privacy policy|Modern Slavery Act Transparency Statement|Sitemap