Neural network for mining implicit relationship between features based on short connection
A neural network and short-connection technology, applied to biological neural network models, neural architectures, instruments, etc., can solve the problems of not making full use of historical features, ignoring feature transferability and reusability, etc.
- Summary
- Abstract
- Description
- Claims
- Application Information
AI Technical Summary
Problems solved by technology
Method used
Image
Examples
Embodiment Construction
[0061] A neural network for mining implicit relationships between features based on short connections of the present invention will be described in detail below with reference to the embodiments and drawings.
[0062] The present invention is a neural network based on short connections to mine the implicit relationship between features, which is an analysis and prediction method based on the neural network-interactive neural network under the background of sparse data. The network model structure mainly includes: 1) a hybrid embedding method that converts sparse sample vectors in the feature space into basic dense sample vectors; 2) a pooling layer based on short connections, which uses historical features to better capture features 3) A neural network based on layer-by-layer feature extraction, which can obtain more meaningful high-level information by modeling the relationship between the historical features contained in each hidden layer and the predicted target. order inte...
PUM
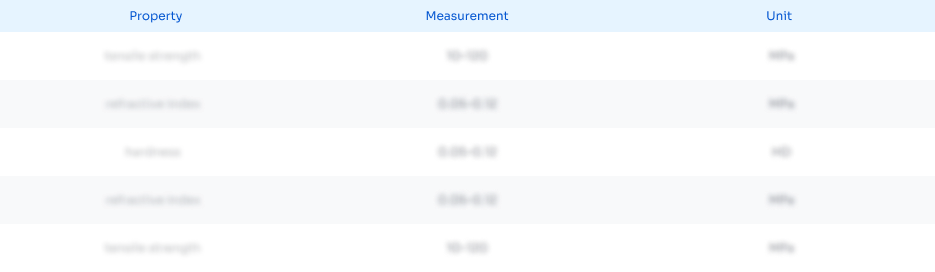
Abstract
Description
Claims
Application Information
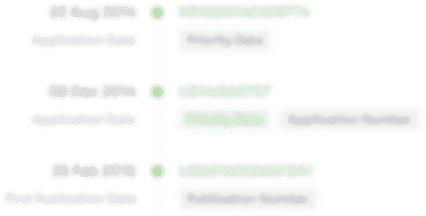
- R&D
- Intellectual Property
- Life Sciences
- Materials
- Tech Scout
- Unparalleled Data Quality
- Higher Quality Content
- 60% Fewer Hallucinations
Browse by: Latest US Patents, China's latest patents, Technical Efficacy Thesaurus, Application Domain, Technology Topic, Popular Technical Reports.
© 2025 PatSnap. All rights reserved.Legal|Privacy policy|Modern Slavery Act Transparency Statement|Sitemap|About US| Contact US: help@patsnap.com