Ultra-short-term power load prediction method
A technology of power load and forecasting method, which is applied in the field of ultra-short-term power load forecasting, which can solve problems such as exponential increase in network complexity, decline in forecasting accuracy, and decrease in forecasting accuracy, so as to improve forecasting accuracy, enhance stability, and reduce training data. volume effect
- Summary
- Abstract
- Description
- Claims
- Application Information
AI Technical Summary
Problems solved by technology
Method used
Image
Examples
Embodiment Construction
[0019] refer to figure 1 , an ultra-short-term load forecasting method provided by the present invention, which combines the random distributed embedding (RDE) framework and BP neural network, and includes steps such as determining the embedding dimension, selecting the number of random embeddings, grouping forecasting, and estimating the final forecast value , which includes the following steps:
[0020] 1) For the active power P of electric load and its influencing factors X 1 ,X 2 ,...,X n Sampling at equal intervals, n∈[1,1000], the sampling interval is τ, τ∈[0.1,100] minutes, the sampling time is t, t-τ, t-2×τ, ..., t-(m-1 )×τ, m∈[100,100000], get (n+1)×m sampling values: X 1 (t-M×τ),X 2 (t-M×τ),…,X n (t-M×τ), P(t-M×τ), where M=0,1,2,...,m-1;
[0021] 2) For the active power samples P(t-M×τ) of m electric loads, use the pseudo-nearest neighbor method to estimate the box-counting dimension d of the electric load system, and determine the embedding dimension D, where...
PUM
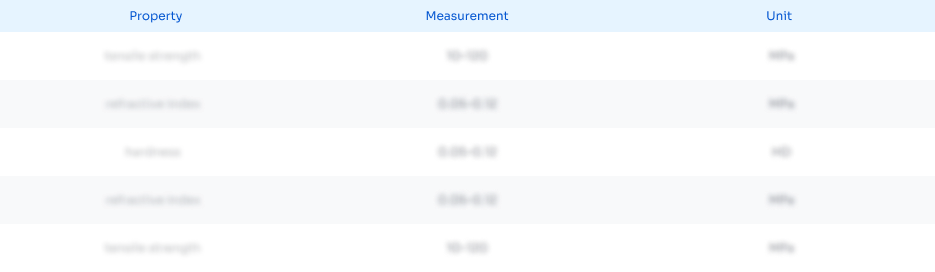
Abstract
Description
Claims
Application Information
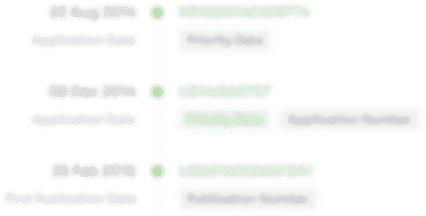
- R&D Engineer
- R&D Manager
- IP Professional
- Industry Leading Data Capabilities
- Powerful AI technology
- Patent DNA Extraction
Browse by: Latest US Patents, China's latest patents, Technical Efficacy Thesaurus, Application Domain, Technology Topic, Popular Technical Reports.
© 2024 PatSnap. All rights reserved.Legal|Privacy policy|Modern Slavery Act Transparency Statement|Sitemap|About US| Contact US: help@patsnap.com