Urban water consumption prediction method based on GA-BP neural network
A BP neural network, GA-BP technology, applied in the field of urban water consumption prediction based on GA-BP neural network, can solve problems such as too fast convergence speed
- Summary
- Abstract
- Description
- Claims
- Application Information
AI Technical Summary
Problems solved by technology
Method used
Image
Examples
Embodiment 1
[0088] refer to Figure 1-5 , an urban water consumption prediction method based on GA-BP neural network, the GA-BP water consumption prediction algorithm includes:
[0089] Step 1. Construction of BP neural network structure:
[0090] 1.1 Construct BP neural network: Determine the number of nodes in the network layer according to the influencing factors and forecasted amount of daily water consumption. The input of the forecasting model is the time series of daily water consumption, and the output of the forecasting model is the hourly water consumption in the future period. The input layer constructed Both the variables of the input layer and the output layer are water consumption, the number of input layer nodes is 24, that is, the water consumption in 24 hours a day, and the output node is the water consumption at a certain time of the next day, so the number of input and output nodes of the neural network is determined to be 24 and 1 respectively ;
[0091] 1.2 Determin...
Embodiment 2
[0153] The simulation is carried out on a PC with Inter(R) Core(TM) i5-4590T CPU@2.00GHz, 4G memory, Windows 7 Ultimate 64-bit system, and the running platform is MATLAB 2015b. The model structure of the GA-BP water consumption prediction algorithm is 24-9-1, the expected error is set to E=0.005, and the maximum number of training times is r=5000. The population size of the genetic algorithm is 50, the crossover probability is 0.4, the mutation probability is 0.1, and the number of iterations is 100 generations.
[0154] Use the GA-BP water consumption prediction algorithm to predict the urban water consumption, select the water consumption data for a week from April 16 to 22 as a training sample, and use the sample data to train the BP water consumption prediction model and the GA-BP water consumption prediction model to predict Daily water consumption of residents in the coming week. The model has been trained many times (the maximum number of training r=5000), and the outp...
Embodiment 3
[0166] For the urban construction of Xi'an, the water supply system combines the water resources of the water plant, the pipe network model and the water consumption of users, and rationally allocates water resources from the perspectives of system-wide safety and reliability, economic operation and water supply feasibility to achieve the overall optimal system , more suitable for the existing Xi'an water supply system. Through the analysis of the optimal scheduling model of Xi’an’s water supply system, and according to the actual water supply situation, the optimal scheduling model of water supply is established. On the basis of satisfying the water consumption and pressure of citizens, the water pressure and flow of the water supply factory are optimized, and the goal of energy saving optimization is obtained.
[0167] The actual outlet flow of the water plant is compared with the outlet flow of the water plant at this moment, which is optimized under the constraint condition...
PUM
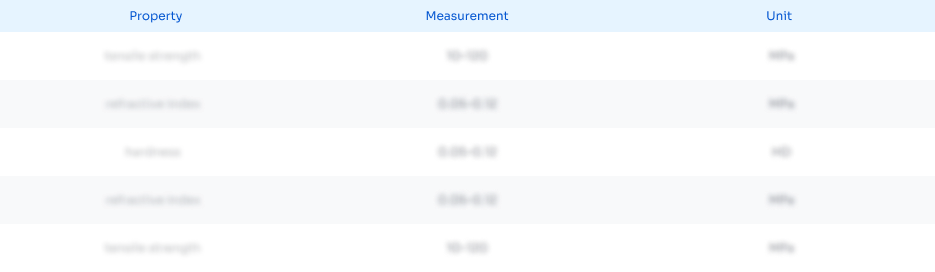
Abstract
Description
Claims
Application Information
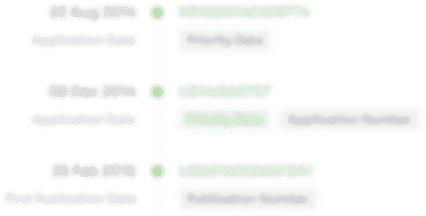
- R&D Engineer
- R&D Manager
- IP Professional
- Industry Leading Data Capabilities
- Powerful AI technology
- Patent DNA Extraction
Browse by: Latest US Patents, China's latest patents, Technical Efficacy Thesaurus, Application Domain, Technology Topic, Popular Technical Reports.
© 2024 PatSnap. All rights reserved.Legal|Privacy policy|Modern Slavery Act Transparency Statement|Sitemap|About US| Contact US: help@patsnap.com