Malicious software classification method and system based on dual-channel convolutional neural network
A convolutional neural network and malware technology, applied in the field of malware detection, can solve problems such as large data volume, cumbersome operation, and unsatisfactory recognition accuracy, and achieve strong applicability
- Summary
- Abstract
- Description
- Claims
- Application Information
AI Technical Summary
Problems solved by technology
Method used
Image
Examples
Embodiment Construction
[0029] The present invention will be further described in detail below in conjunction with the accompanying drawings and specific embodiments.
[0030] The invention discloses a malware classification method and system based on a double-channel convolutional neural network.
[0031] In order to accurately and effectively detect and classify software, the present invention selects the operation code sequence and sensitive API features of the application program as detection features. The present invention comprehensively considers when selecting features: 1) the effectiveness of features, that is, whether the features can effectively distinguish malware from non-malware; 2) the degree of automation of feature extraction, which is to cope with the emergence of automatic malware generators ; 3) The spatio-temporal efficiency of feature detection, that is, the selected features should not be too resource-intensive.
[0032] Such as figure 1 Shown, the malicious software classifi...
PUM
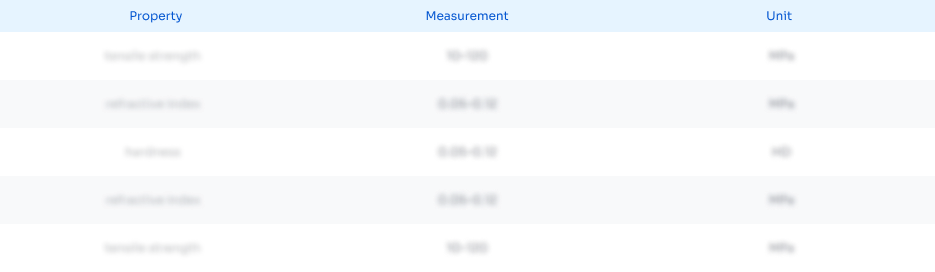
Abstract
Description
Claims
Application Information
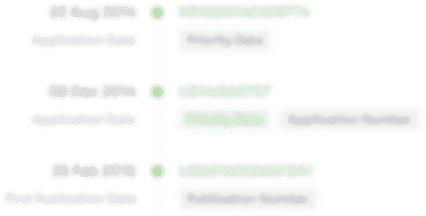
- R&D
- Intellectual Property
- Life Sciences
- Materials
- Tech Scout
- Unparalleled Data Quality
- Higher Quality Content
- 60% Fewer Hallucinations
Browse by: Latest US Patents, China's latest patents, Technical Efficacy Thesaurus, Application Domain, Technology Topic, Popular Technical Reports.
© 2025 PatSnap. All rights reserved.Legal|Privacy policy|Modern Slavery Act Transparency Statement|Sitemap|About US| Contact US: help@patsnap.com