Fine-grained image classification method based on generative adversarial network and attention network
A classification method and attention technology, applied in the field of image processing, can solve the problems of large overall calculation, not explaining the principle of feature area acquisition, and not being well compatible with the whole.
- Summary
- Abstract
- Description
- Claims
- Application Information
AI Technical Summary
Problems solved by technology
Method used
Image
Examples
Embodiment Construction
[0036] The present invention will be described in further detail below through examples, and the following examples are explanations of the present invention and the present invention is not limited to the following examples.
[0037] A fine-grained image classification method based on generative confrontation network and attention network, including the following steps:
[0038] Step 1: Determine the image classification category, and establish a training image set of the corresponding category.
[0039] 1.1 Determine the list of image categories to be classified.
[0040] 1.2 Create an image folder for each category, collect images containing the target in the folder, and basically ensure that the number of image samples for each category is at least 10,000.
[0041] 1.3 Use the method of target detection to detect the position of the target from the image, and segment each subcategory image from the global image based on the position.
[0042] Step 2: Design a deep attent...
PUM
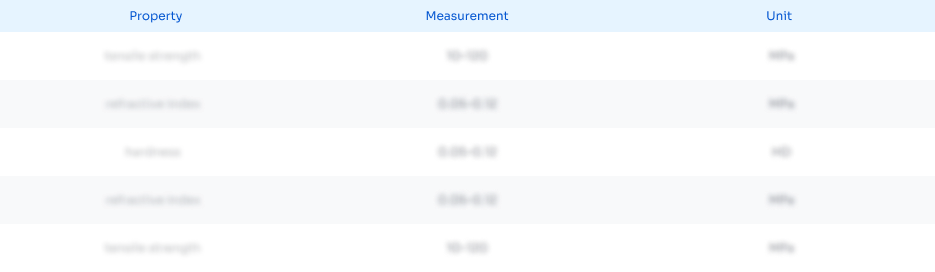
Abstract
Description
Claims
Application Information
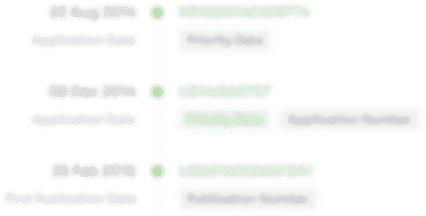
- R&D Engineer
- R&D Manager
- IP Professional
- Industry Leading Data Capabilities
- Powerful AI technology
- Patent DNA Extraction
Browse by: Latest US Patents, China's latest patents, Technical Efficacy Thesaurus, Application Domain, Technology Topic, Popular Technical Reports.
© 2024 PatSnap. All rights reserved.Legal|Privacy policy|Modern Slavery Act Transparency Statement|Sitemap|About US| Contact US: help@patsnap.com