Bearing fault diagnosis method based on semi-supervised generative adversarial network
A fault diagnosis, semi-supervised technology, applied in biological neural network models, neural learning methods, testing of mechanical components, etc., can solve problems such as time-consuming and labor costs, and achieve convenient operation, good diagnosis effect and noise resistance. , compact structure
- Summary
- Abstract
- Description
- Claims
- Application Information
AI Technical Summary
Problems solved by technology
Method used
Image
Examples
Embodiment
[0088]Take the dataset provided by the publicly available Case Western Reserve University Bearing Data Center website as an example
[0089] 1. Diagnosis process based on one-dimensional semi-supervised generative confrontation network
[0090] The bearing model is SKF6205, the rotational speed is 1797rpm, the damage locations are the inner ring, the rolling element, and the outer ring, and the damage diameters are 0.007, 0.014, and 0.021 inches respectively. There are 9 fault states and 1 normal state in total; the vibration signal collection frequency is 12kHz, so the sampling points obtained by one rotation of the bearing are about 400, the calculation is as follows:
[0091]
[0092] In the inner circle where the damage diameter is 0.007 inches, 121,991 sampling points are collected by the vibration signal acquisition equipment; then select the first 120,000 sampling points, and take 400 sampling points as a sample, then a total of 300 samples; according to 9: The rati...
PUM
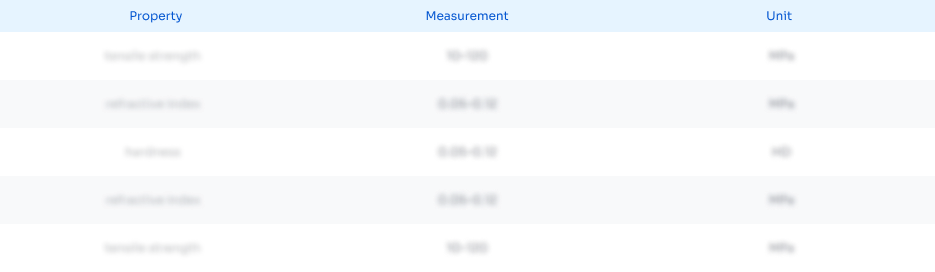
Abstract
Description
Claims
Application Information
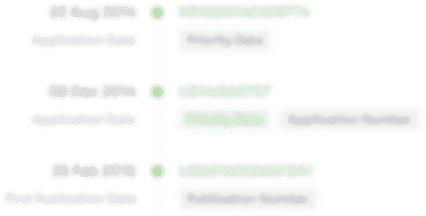
- R&D
- Intellectual Property
- Life Sciences
- Materials
- Tech Scout
- Unparalleled Data Quality
- Higher Quality Content
- 60% Fewer Hallucinations
Browse by: Latest US Patents, China's latest patents, Technical Efficacy Thesaurus, Application Domain, Technology Topic, Popular Technical Reports.
© 2025 PatSnap. All rights reserved.Legal|Privacy policy|Modern Slavery Act Transparency Statement|Sitemap|About US| Contact US: help@patsnap.com