Rotating machinery fault diagnosis method based on multi-attention convolution neural network
A convolutional neural network, rotating machinery technology, applied in neural learning methods, biological neural network models, neural architectures, etc., can solve problems such as lack of sufficient research results, waste of resources, failure to learn features related to failures, etc. Fault diagnosis performance, reinforcement learning, effect of suppressing irrelevant noise
- Summary
- Abstract
- Description
- Claims
- Application Information
AI Technical Summary
Problems solved by technology
Method used
Image
Examples
Embodiment
[0040] figure 1 It is a flowchart of a specific embodiment of the method for diagnosing faults of rotating machinery based on multi-attention convolutional neural networks in the present invention. Such as figure 1 As shown, the specific steps of the rotating machinery fault diagnosis method based on multi-attention convolutional neural network of the present invention include:
[0041] S101: Collect vibration signal samples of rotating machinery:
[0042] at sampling frequency f s Acquisition of acceleration vibration signals of non-faulty and different faulty rotating machinery under different operating conditions x m [n], where m=1,2,...,M, M represents the quantity of the acceleration vibration signal collected, n=1,2,...,N, N represents the number of sampling points in each acceleration vibration signal, thus Get acceleration vibration signal set X={x 1 [n],x 2 [n],...,x M [n]}. And according to each acceleration vibration signal x m [n] corresponds to the fault ...
PUM
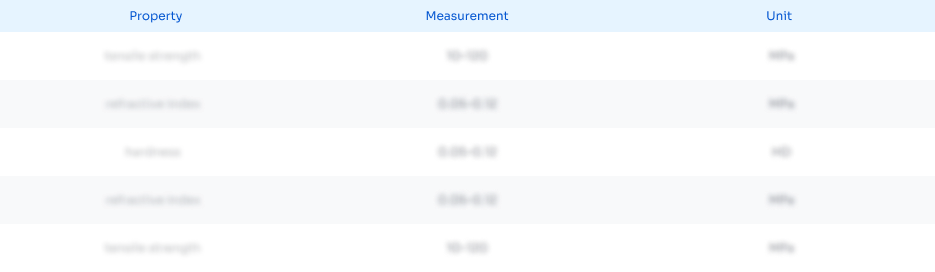
Abstract
Description
Claims
Application Information
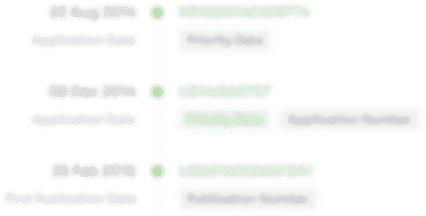
- R&D Engineer
- R&D Manager
- IP Professional
- Industry Leading Data Capabilities
- Powerful AI technology
- Patent DNA Extraction
Browse by: Latest US Patents, China's latest patents, Technical Efficacy Thesaurus, Application Domain, Technology Topic, Popular Technical Reports.
© 2024 PatSnap. All rights reserved.Legal|Privacy policy|Modern Slavery Act Transparency Statement|Sitemap|About US| Contact US: help@patsnap.com