Method and device of recognizing ventricular tachycardia heart rhythm based on transfer learning
A technology of ventricular tachycardia and transfer learning, which is applied in medical science, sensors, diagnostic recording/measurement, etc., can solve the problems of insufficient recognition accuracy of ventricular tachycardia heart rhythm, and achieve high accuracy and recognition accuracy high effect
- Summary
- Abstract
- Description
- Claims
- Application Information
AI Technical Summary
Problems solved by technology
Method used
Image
Examples
Embodiment
[0106] This embodiment provides a ventricular tachycardia rhythm recognition method based on transfer learning, comprising the following steps:
[0107] S1: Obtain multi-lead ECG signals, such as 12-lead signals;
[0108] S2: Input the multi-lead ECG signal into the SCNN neural network with several convolutional layers, several pooling layers and several fully connected layers whose output value is [X, Y] trained based on transfer learning, the simplest Processing can directly set X to 0 and Y to 1;
[0109] S3: If the output result of the SCNN neural network is greater than or equal to (X+Y) / 2, X is set to 0 and Y is set to 1 and greater than or equal to 0.5, then the type of the multi-lead ECG signal is considered to be ventricular Otherwise, it is considered that the type of the multi-lead ECG signal is non-ventricular tachycardia.
[0110] In the S2 step, the following steps are included when the SCNN neural network is trained:
[0111]S21: Collect enough (not less than...
Embodiment approach
[0116] As a specific implementation, the SCNN neural network includes fifteen convolutional layers, fifteen pooling layers, and two fully connected layers;
[0117] Among them, the first layer is a convolutional layer, which contains 12 filters, the convolution kernel size is (121,12), the step size is 1, and the activation function is leakyReLU;
[0118] The second layer is the pooling layer, the pooling window size is 2, and the pooling method is used to maximize the pooling;
[0119] The third layer is the convolution layer, which contains 10 filters, the convolution kernel size is (91,12), the step size is 1, and the activation function is leakyReLU;
[0120] The fourth layer is the pooling layer, the pooling window size is 2, and the maximum pooling method is used for pooling;
[0121] The fifth layer is the convolution layer, which contains 8 filters, the convolution kernel size is (71,10), the step size is 1, and the activation function is leakyReLU;
[0122] The sixt...
PUM
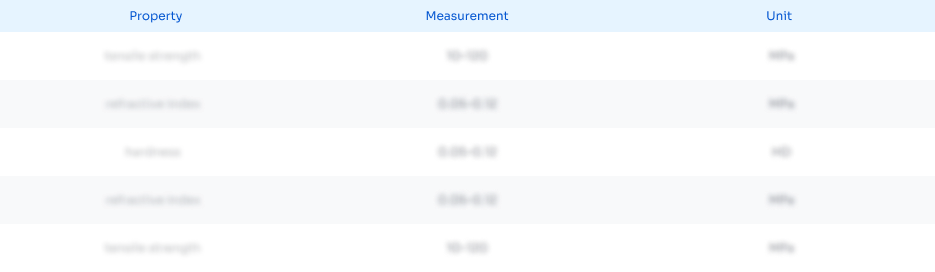
Abstract
Description
Claims
Application Information
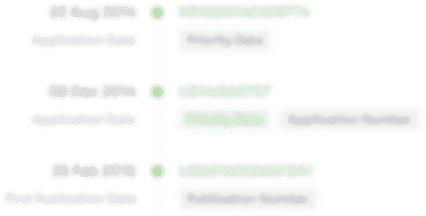
- R&D Engineer
- R&D Manager
- IP Professional
- Industry Leading Data Capabilities
- Powerful AI technology
- Patent DNA Extraction
Browse by: Latest US Patents, China's latest patents, Technical Efficacy Thesaurus, Application Domain, Technology Topic.
© 2024 PatSnap. All rights reserved.Legal|Privacy policy|Modern Slavery Act Transparency Statement|Sitemap