Rolling bearing fault diagnosis method based on improved variational mode decomposition and extreme learning machine
A variational modal decomposition and extreme learning machine technology, applied in mechanical bearing testing, computational models, biological models, etc., can solve the problems of modal aliasing, large influence of signal decomposition, and long time to establish diagnostic models.
- Summary
- Abstract
- Description
- Claims
- Application Information
AI Technical Summary
Problems solved by technology
Method used
Image
Examples
Embodiment Construction
[0049] The specific embodiments of the present invention will be described in detail below with reference to the accompanying drawings, but the present invention is not limited by the specific embodiments.
[0050] The example of the present invention uses the rolling bearing fault signal as the experimental basis for analysis and processing. The bearing model 6205-2RS JEM SKF, the number of rolling elements is 9, and the single-point fault with a diameter of 0.1778mm and a depth of 0.2794mm is artificially introduced into the bearing through EDM technology. The vibration acceleration signals of the rolling bearing under different single fault types are collected through the acceleration vibration sensors installed in different parts of the rolling bearing. The sampling frequency is 12kHz and the speed is 1797rpm. The data contains 4 types of rolling bearing fault data, including normal data and inner ring fault data. , Outer ring fault data, rolling element fault data, each type ...
PUM
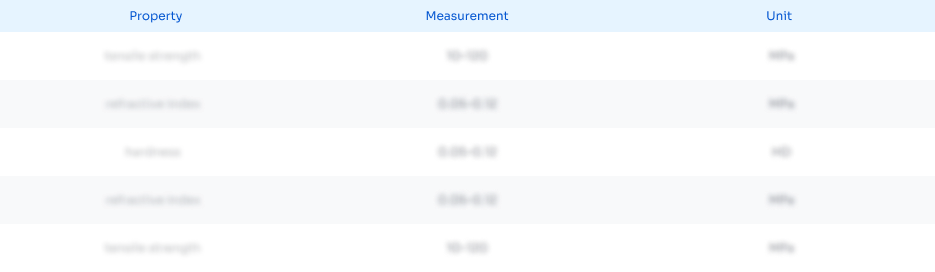
Abstract
Description
Claims
Application Information
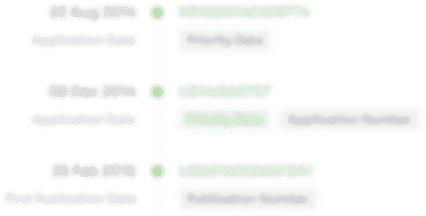
- R&D
- Intellectual Property
- Life Sciences
- Materials
- Tech Scout
- Unparalleled Data Quality
- Higher Quality Content
- 60% Fewer Hallucinations
Browse by: Latest US Patents, China's latest patents, Technical Efficacy Thesaurus, Application Domain, Technology Topic, Popular Technical Reports.
© 2025 PatSnap. All rights reserved.Legal|Privacy policy|Modern Slavery Act Transparency Statement|Sitemap|About US| Contact US: help@patsnap.com