Prediction method and system for popularity values of different cities and model training method and system
A technology of model training and popularity value, applied in the field of computer information, can solve the problem that the popularity of different cities cannot be effectively predicted, and achieve the effect of good housing status and inventory optimization
- Summary
- Abstract
- Description
- Claims
- Application Information
AI Technical Summary
Problems solved by technology
Method used
Image
Examples
Embodiment 1
[0077] This embodiment provides a model training method for travel data prediction, figure 1 A flowchart of the model training method is shown, including:
[0078] Step 101. Obtain historical travel data about multiple target cities from the OTA platform.
[0079] Step 102, extract feature data from the historical travel data.
[0080] Step 103. Divide the feature data into a training set and a prediction set.
[0081] Step 104, input the feature data in the training set into the deep machine learning model for training.
[0082] Step 105, input the feature data in the prediction set into the trained deep machine learning model for prediction to obtain predicted travel data.
[0083] Step 106 , judging whether the relative error between the predicted travel data and the real travel data is smaller than the error threshold, if yes, execute step 107 , if not, return to step 104 .
[0084] Step 107, confirming that the trained deep machine learning model is a travel data pred...
Embodiment 2
[0090] This embodiment provides a model training method for travel data prediction. This embodiment is an improvement to Embodiment 1, wherein, in order to fully learn the training set in the deep machine learning model, effective features can be extracted. , and can also overcome the situation of overfitting and data leakage. The deep machine learning model in this embodiment includes two layers of machine learners, and the output of the first layer of machine learners is used as the input of the second layer of machine learners. Among them, the first layer of machine learning includes several models so that the data set can be divided and cyclically trained to reduce the risk of overfitting. For example, 5-fold cross-validation can be used, including Xgboost model, LightGBM model, BayesianRidge model, DecisionTreeRegressor model and The RandomForestRegressor model, the second layer machine learner includes five models of the Xgboost model. Among them, since the Xgboost model...
Embodiment 3
[0096] This embodiment provides a method for predicting heat values of different cities, image 3 A flowchart of the forecasting method is shown, including:
[0097] Step 301: Input the city to be predicted into the travel data prediction model to obtain the daily travel data of the city to be predicted within the preset range of future dates.
[0098] Step 302: Input the travel data into a preset mapping function to obtain the popularity level of the city to be predicted.
[0099] Wherein, the travel data prediction model is trained according to the training method of embodiment 1 or embodiment 2. In this embodiment, the trained travel data prediction model can be deployed to online scheduling for predicting the cities that need to be predicted, and When prediction is required, the travel data prediction model can be invoked by loading an online model file.
[0100] Wherein, in one implementation manner of this embodiment, the predicted travel data is arrival number data,...
PUM
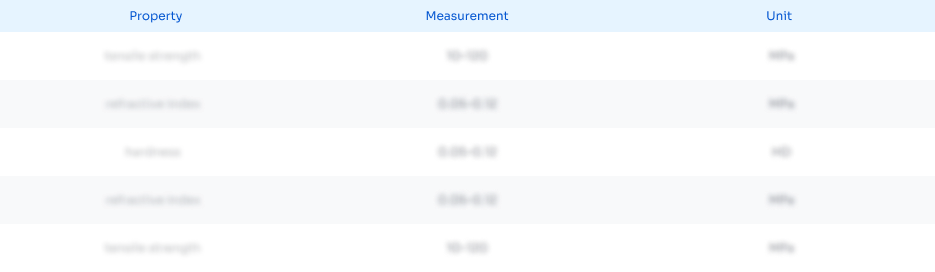
Abstract
Description
Claims
Application Information
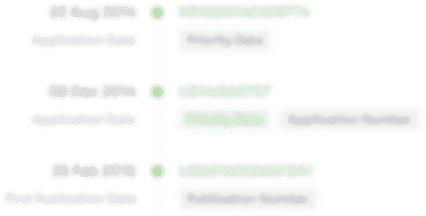
- R&D Engineer
- R&D Manager
- IP Professional
- Industry Leading Data Capabilities
- Powerful AI technology
- Patent DNA Extraction
Browse by: Latest US Patents, China's latest patents, Technical Efficacy Thesaurus, Application Domain, Technology Topic, Popular Technical Reports.
© 2024 PatSnap. All rights reserved.Legal|Privacy policy|Modern Slavery Act Transparency Statement|Sitemap|About US| Contact US: help@patsnap.com