Token-based multi-class anomaly detection method
An anomaly detection and multi-category technology, applied in character and pattern recognition, instruments, biological neural network models, etc., can solve problems such as failure to function, failure to output probability, low precision, etc., to reduce the complexity of decision-making space and simplify the process , the effect of improving efficiency
- Summary
- Abstract
- Description
- Claims
- Application Information
AI Technical Summary
Problems solved by technology
Method used
Image
Examples
Embodiment Construction
[0024] In order to enable those skilled in the art to better understand the solutions of the present invention, the technical solutions in the embodiments of the invention will be clearly and completely described below in conjunction with the drawings in the embodiments of the present invention. Obviously, the described embodiments are only It is a part of embodiments of the present invention, but not all embodiments. Based on the embodiments of the present invention, all other embodiments obtained by persons of ordinary skill in the art without making creative efforts shall fall within the protection scope of the present invention.
[0025] A Token-based multi-category anomaly detection method, comprising the following steps:
[0026] 1) Obtain the data source to be analyzed;
[0027] 2) Training data set, test data set data source vectorization
[0028] The data source type of abnormal machine is digital, such as sound, vibration, temperature, sensor, and logfile records o...
PUM
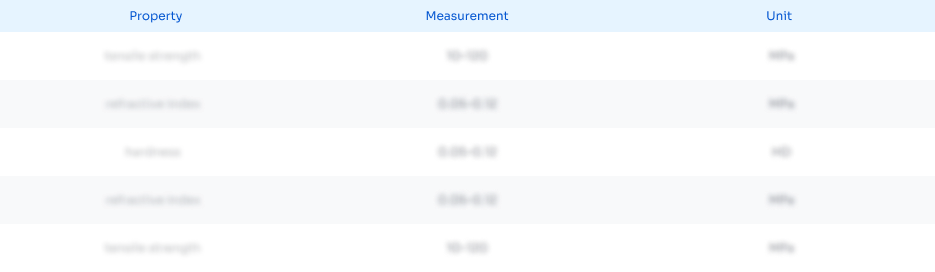
Abstract
Description
Claims
Application Information
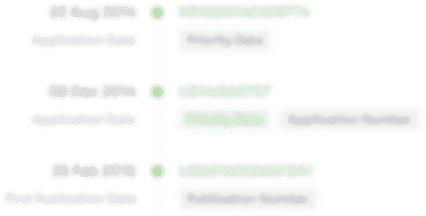
- R&D
- Intellectual Property
- Life Sciences
- Materials
- Tech Scout
- Unparalleled Data Quality
- Higher Quality Content
- 60% Fewer Hallucinations
Browse by: Latest US Patents, China's latest patents, Technical Efficacy Thesaurus, Application Domain, Technology Topic, Popular Technical Reports.
© 2025 PatSnap. All rights reserved.Legal|Privacy policy|Modern Slavery Act Transparency Statement|Sitemap|About US| Contact US: help@patsnap.com